Dear friends,
When I was younger, I was not a fan of working from home. Too many distractions! So I worked a lot in coffee shops. They turned out to be convenient places to talk to strangers and ask for feedback about products I was working on, including early MOOC prototypes.
Now much of the world is undergoing a remote work experiment. My teams and I are working from home.
There have been positives and negatives. I love running into colleagues in our #virtualcoffeechat slack channel, especially people I don’t see so often around the office. I love reducing my carbon footprint and not having to commute, and I love getting to see Nova during my lunch break. (She’s learning to walk, and her unstable toddling is simultaneously cute and terrifying.)
On the flip side, I miss seeing everyone in 3D. I miss the serendipitous discussions, and I miss being able to gather in the break room to chat and partake in the babka, gulab jamun, chicharron, and durian candy that teammates sometimes bring to share.
Even though Covid-19 is a painful challenge, there is a silver lining in this shift in how we work. People in the tech industry are fortunate that a lot of work can be done remotely, and many companies are now learning how to do this well.
Once this pandemic is over, I believe that many remote roles will open up. It will be easier for an aspiring AI engineer who lives in Dallas to get a job in Silicon Valley — without having to move. A recruiter who lives in Buenos Aires will have a better chance of being hired by a company in Montreal. A front-end engineer in Sydney might work for an employer in Tokyo. No matter where you live, more jobs will be coming to you in the future.
Stay safe and keep learning!
Andrew
News
Satellite Data Hints at China Upswing
Neural networks revealed both how hard Covid-19 has hit the Chinese economy, and hopeful signs that a renaissance may be underway. What’s new: Researchers at WeBank, a Chinese financial institution, analyzed satellite imagery, GPS signals, and social media to get a multifaceted view of the pandemic's impact. What they found: The team compared data collected before, during, and after the peak of China’s containment efforts. It focused on three data sources:
- Satellite images: The researchers adapted SolarNet, an image recognition model that maps solar panels based on infrared satellite photos, to look for heat signatures from steel mills. Then they correlated the results with steel output. In late January, at the height of the outbreak, China’s steel production had dropped to 30 percent of capacity, they found. By February 9, it had recovered to 76 percent.
- GPS: Using a different model, the researchers analyzed anonymized GPS signals collected in 2019 from millions of phones to determine whether they belonged to commuters. They used the results to estimate economic activity. Then they analyzed signals surrounding the quarantines to assess the impact. Comparing the datasets, they found that the country's economic activity had fallen to 20 percent of normal by February 9. A month later, however, it had rebounded to 72 percent. Extrapolating the findings, they predict that China's economy will recover fully by March 26.
- Social media: The researchers used natural language processing to scan social media posts to determine whether people were working from homes or offices. According to that analysis, the number of telecommuters ballooned by more than sixfold between January 1 and mid-March.
Behind the news: China dramatically cut its coronavirus transmission rate by imposing strict measures to limit social interaction including a quarantine of 50 million people in the province that includes Wuhan, the disease’s epicenter. Why it matters: Before Covid-19 rocked China’s economy, J.P. Morgan had estimated that the country’s GDP would grow nearly 6 percent by the end of 2020. Now the U.S. investment bank predicts a meager 1 percent growth. China’s isn’t the only economy in trouble: The bank's analysts warn of a global recession. We’re thinking: China’s economic revival would be great news for the rest of the world. But full recovery isn’t likely until its Western trading partners come back up to speed.
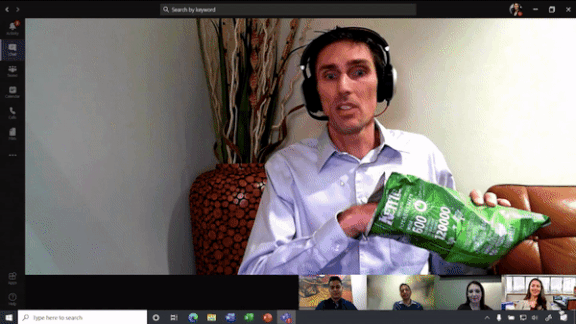
Silent Snacking
As working from home becomes the new normal, AI may protect you from the sound of coworkers munching while they chat. What’s new: No more smacking lips and rustling chip bags! Microsoft’s online collaboration platform Teams announced a feature that removes extraneous sounds from videoconferences. How it works: The Teams team trained neural networks to recognize and filter out non-speech noises using datasets they built for the 2020 Deep Noise Suppression Challenge.
- The researchers curated 500 hours worth of 30 second clips from a repository of public-domain audiobooks.
- They combined half of this set with 60,000 annotated clips from YouTube videos. Those files represented 150 different classes of noise including hours of crinkling and chewing.
- A recurrent neural net learned the difference between voices overlaid with noise and their clean counterparts.
- Microsoft expects to make the feature available later this year.
Behind the news: People across the globe are hunkering down for a long virus season. Zoom added more than 2 million monthly active users in January and February, more than in all of 2019. Microsoft Teams’ daily user count shot up from 13 million to 44 million between July 2019 and March 2020. Slack, the other big telecommuting program, hasn’t published monthly average user numbers since October, when the tally was 12 million. Why it matters: Nobody wants to listen to your mukbang during working hours. We’re thinking: Next, can we get a feature that filters out intrusive toddlers?
Deep Learning Finds New Antibiotic
Chemists typically develop new antibiotics by testing close chemical relatives of tried-and-true compounds like penicillin. That approach becomes less effective, though, as dangerous bacteria evolve resistance to those very chemical structures. Instead, researchers enlisted neural networks. What’s new: Jonathan Stokes and colleagues at MIT, Harvard, and McMaster University built an ensemble model that predicts molecules that are structurally unrelated to known antibiotics, harmless to humans, and deadly to E. coli, a common bacterium that served as a proxy microorganism. The model spotted a previously unrecognized antibiotic that proved effective at killing a variety of germs. Key insight: Neural networks can stand in for petri dishes to zero in on promising molecules. An initial simulation reduced an enormous number of molecules to a few thousand solid possibilities, of which the model selected a couple dozen for testing in a wet lab. How it works: The researchers used an ensemble of 20 graph neural networks (GNNs) to evaluate molecules’ ability to inhibit E. coli, and another ensemble of five GNNs to evaluate toxicity. They used a standard measure to evaluate chemical structure. Then they tested the most promising compounds on mice.
- Each GNN examines molecules atom by atom. The Chemprop architecture learns a vector for each atom based on its atomic number, mass, other properties along with vectors of the atoms it’s bound to.
- The GNN graphs collapse into vectors that describe the molecule as a whole.
- Fully connected layers predict either E. coli inhibition based on labels from an FDA library or toxicity based on a dataset of qualitative evaluations of approved drugs.
Results: The researchers examined more than 107 million compounds to produce a ranked list. Empirical tests on the top-ranked 3,260 chemicals yielded 51 that were effective. Of those, 23 had low predicted toxicity and structures distinct from known antibiotics. In mouse experiments, Halicin, a known diabetes treatment, proved effective as a broad-spectrum antibiotic. Why it matters: Alexander Fleming’s discovery of penicillin in 1928 revolutionized medicine. Now that transformation is at risk as bugs evolve resistance to that drug and its successors. Discovery of new antibiotics has been hampered by lack of a way to narrow the list of possibilities for lab tests. This method offers a way to vet candidates quickly and efficiently.
We’re thinking: Antibiotic-resistant bugs are responsible for 2.8 million infections and 35,000 deaths annually in the U.S. alone. Crank up those GNNs!
A MESSAGE FROM DEEPLEARNING.AI
Get your models running on iOS and Android devices. Take the first step in Course 2 of the TensorFlow: Data and Deployment Specialization. Enroll now
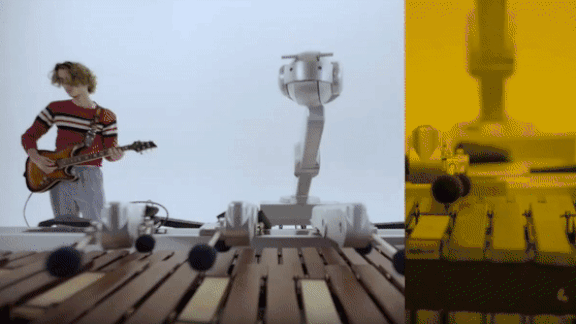
Algorithm and Blues
Bored with your Spotify playlists? Let this robot singer/songwriter take you on a trip “Into Your Mind.” What's Goin' On: A music-composing, marimba-playing robot named Shimon has learned to write and sing its own lyrics, IEEE Spectrum reports. Shimon performs its debut single with a flesh-and-blood backup band in this video. An album is scheduled to drop on Spotify on April 10. (A)I Write the Songs: Two of the robot’s creators, Georgia Tech professor Gil Weinberg and grad student Richard Savery, treat the machine as though it were a human collaborator.
- Shimon’s language model was trained on 50,000 lyrics from jazz, rock, and hip hop songs. Given a keyword, it generates thousands of phrases that are related to the word itself, its synonyms, or its antonyms. It picks a song’s worth of phrases, emphasizing rhymes and thematic similarity.
- After it has completed the lyrics, the humans weave melodies it generates with their own ideas to produce music that suits the words.
- Shimon’s voice is based on a model developed by the University of Pomeu Fabra and trained on human voices in a wide variety of pitches.
- Weinberg aims to combine Shimon’s musical improvisations with its lyrical skills to transform the machine into a freestyle rapper.
Robot Rock: Shimon’s music-video debut showcases several other innovations created by Weinberg and his colleagues, who have been working on this project for nearly a decade.
- The robot's vaguely serpentine head automatically moves to the rhythm while its mouth and eyebrows emote along with the lyrics.
- The system originally was designed to improvise on marimba in response to notes played by human musicians. New hardware enables its arms to play more precisely in time and with greater control over loud and soft accents.
- Shimon’s human bandmate Jason Barnes lost one hand in a 2012 accident. He performs on drums using a robotic prosthesis that holds two sticks. Electrical activity from Barnes’ muscle controls one stick, while the second stick moves autonomously in response to the music.
Unchained Melody: Machine learning is challenging timeless assumptions about human creativity. Shimon’s output is reminiscent of progressive rock masters. Could it conquer the pop charts by working with the producers of Taylor Swift, Beyonce, or Drake? Wish You Were Here: The Covid-19 pandemic forced Weinberg to postpone plans to take Shimon on tour. Will his next project be building robot fans?
Rightsizing Neural Nets
How much data do we want? More! How large should the model be? Bigger! How much more and how much bigger? New research estimates the impact of dataset and model sizes on neural network performance. What’s new: Jonathan Rosenfeld and colleagues at MIT, York University, Harvard, Neural Magic, and Tel Aviv University introduced an equation — a so-called error landscape — that predicts how much data and how large a model it takes to generalize well. Key insight: The researchers made some assumptions; for instance, models without training should be as accurate as a random guess. They combined these assumptions with experimental observations to create an equation that works for a variety of architectures, model sizes, data types, and dataset sizes. How it works: The researchers trained various state-of-the-art vision and language models on a number of benchmark datasets. Considering 30 combinations of architecture and dataset, they observed three effects when varying data and model size:
- For a fixed amount of data, increasing model size initially boosted performance on novel data, though effect leveled off. The researchers observed a similar trend as they increased the amount of training data. The effect of boosting both model and dataset size was approximately the same as the combined impact of changing each one individually.
- An equation captures these observations. It describes the error as a function of model and data size, forming a 3D surface or error landscape.
- The equation contains variables dependent on the task. Natural language processing, for instance, often requires more data than image processing. A simple regression can determine their values for a target dataset.
Results: After fitting dataset-specific variables to the validation dataset, the researchers compared the predicted model error against the true error on the test set. The predictions were within 1 percent of the true error, on average. Why it matters: It turns out that the impact on accuracy of model and dataset size is predictable. How nice to have an alternative to trial and error! Yes, but: When varying network sizes, the researchers focused mainly on scaling width while holding the rest of the architecture constant. Neural network “size” can't be captured in a single number, and we look forward to future work that considers this nuance. We’re thinking: Learning theory offers some predictions about how algorithmic performance should scale, but we're glad to see empirically derived rules of thumb.
AI's Gender Imbalance
Women continue to be severely underrepresented in AI. What’s new: A meta-analysis of research conducted by Synced Review for Women’s History Month found that female participation in various aspects of AI typically hovers between 10 and 20 percent. What they found: Much of the research included in the analysis was based on numbers generated by rules-based software that categorizes names according to gender. Synced Review, which is based in China, said it didn’t examine studies of Chinese companies or institutions because Chinese names don't correlate as tightly with gender as names in other languages.
- The 2019 AI Index produced by Stanford University’s Human-Centered AI Institute reported that females made up 20 percent of faculty members in academic AI departments. That number isn’t likely to rise soon; 20 percent of new faculty hires and 20 percent of AI-related PhD recipients are female.
- A 2018 study by Wired and Element AI, an enterprise software company, tallied men and women who contributed to the major AI conferences NeurIPS, ICLR, and ICML. Twelve percent were women. In a 2019 review of 21 conferences by Element AI, the percentage rose to 18 percent.
- A 2018 Wired analysis of AI researchers at Google and Facebook estimated that 10 percent of Google’s machine learning workforce and 15 percent of Facebook’s AI researchers were women. (Both companies later said the report had understated the true number, but they didn't provide further information.)
- Researchers at Nesta, a UK research foundation, analyzed AI research papers on Arxiv. Women accounted for 12 percent of authors in 2015, less than in 2009.
Behind the news: Women have a prominent place in AI’s history, going all the way back to Ida Rhodes, who in the 1960s laid the groundwork for natural language processing. The percentage of American women with computer science degrees, however, peaked in the mid-1980s at around 35 percent, and since has declined to under 20 percent. Why it matters: It's important that people building the future represent diverse groups to make sure that anyone can participate and that the products we build encompass a variety of perspectives. We’re thinking: Each one of us can help promote diversity. Leaders can make an effort to interview, hire, and mentor underrepresented groups, and everyone can help make the workplace inclusive.
Work With Andrew Ng
Chief Technology Officer: TalkTime, an AI Fund portfolio company, seeks a CTO. You will be responsible for creating an effective way for customers and companies to interact leveraging AI, natural-language processing, and a mobile-first user interface. Apply here
Senior Full-Stack Engineer in Medellín, Colombia: Bearing AI, an AI Fund portfolio company, is looking for an experienced full-stack engineer. You will be responsible for building out the API to support our web app as well as helping to develop new AI-driven products. Contact our team directly at estephania@aifund.ai or apply here
Front-End Engineer in Medellín, Colombia: Bearing AI is looking for a talented engineer to take end-to-end ownership of our web app and collaborate closely with our designer and back-end engineering team. Contact our team directly at estephania@aifund.ai or apply here
Thoughts, suggestions, feedback? Please send to thebatch@deeplearning.ai. Subscribe here and add our address to your contacts list so our mailings don't end up in the spam folder. You can unsubscribe from this newsletter or update your preferences here.
Copyright 2020 deeplearning.ai, 195 Page Mill Road, Suite 115, Palo Alto, California 94306, United States. All rights reserved.
|