Dear friends,
Last Sunday was my birthday. That got me thinking about the days leading to this one and those that may lie ahead.
As a reader of The Batch, you’re probably pretty good at math. But let me ask you a question, and please answer from your gut, without calculating.
How many days is a typical human lifespan?
-
20,000 days
-
100,000 days
-
1 million days
-
5 million days
When I ask friends, many choose a number in the hundreds of thousands. (Many others can’t resist calculating the answer, to my annoyance!)
When I was a grad student, I remember plugging my statistics into a mortality calculator to figure out my life expectancy. The calculator said I could expect to live a total of 27,649 days. It struck me how small this number is. I printed it in a large font and pasted it on my office wall as a daily reminder.
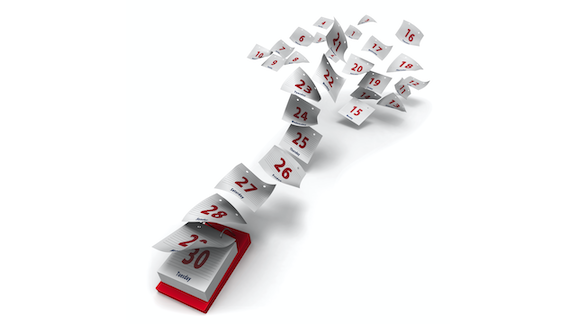
That’s all the days we have to spend with loved ones, learn, build for the future, and help others. Whatever you’re doing today, is it worth 1/30,000 of your life?
Let’s make every day count.
Keep learning!
Andrew
P.S. Don’t worry about me. I’m healthy and plan to stick around for awhile.
P.P.S. A huge thank-you to everyone who responded to my earlier online note about my birthday! ❤️
News
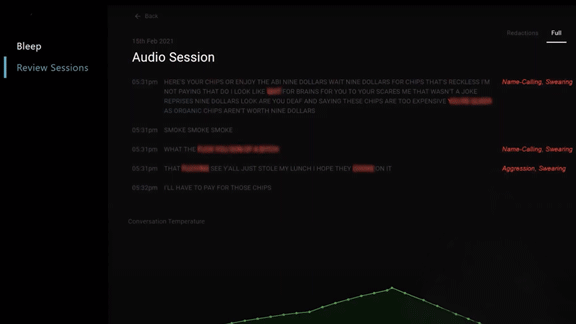
Haters Gonna [Mute]
A new tool aims to let video gamers control how much vitriol they receive from fellow players.
What’s new: Intel announced a voice recognition tool called Bleep that the company claims can moderate voice chat automatically, allowing users to silence offensive language. The system is in beta-test and scheduled for release later this year.
How it works: Chip maker Intel worked with Spirit AI, which develops technology for content moderation, to let users of voice chat fine-tune how much of specific types of offensive language can reach their ears.
- Bleep combines speech detection technology with Spirit’s flagship product, which determines whether a phrase constitutes harassment in the context of surrounding chatter.
- The system classifies offensive speech in nine categories including misogyny, sexually explicit language, and anti-LGBTQ hate speech. Users can opt to filter out none, some, most, or all content in any category. For a tenth category called N-word, the system offers an on/off switch.
- It runs on Windows PCs and, since it interacts directly with Windows’ audio controls, it can work with a variety of voice-chat apps.
Behind the news: ToxMod also aims to moderate video game voice chat and provides a dashboard for human moderators to track offensive speech across servers. Hive’s system is designed to moderate audio, video, text, and images. Its customers include Chatroulette, which uses Hive’s technology to help users avoid unwanted nudity. Two-Hat’s text-moderation system detects efforts to subvert moderation by, say, intentionally misspelling slurs and other potentially offensive language.
Why it matters: There’s a clear need for tools that help people enjoy networked communications without being targeted by abuse. Twenty-two percent of U.S. online gamers stopped playing certain games after experiencing verbal harassment, according to a survey by the Anti-Defamation League.
We’re thinking: For those whose first thought is, “Censorship!,” note that users will control this auto-moderation capability locally. At the same time, there’s a fine line between blocking harassment and shutting out perspectives we don't currently share. In an ideal world, players would take it upon themselves to keep their conversations civil. Until that day comes, AI will play a valid — if worrisome at times — role.
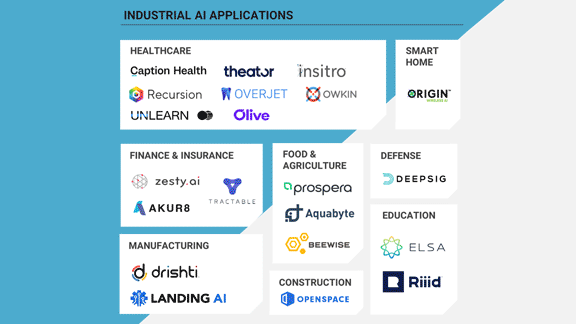
Cream of the Startup Crop
AI startups continue to roared ahead, global pandemic or no.
What’s new: Tech industry analyst CB Insights published its fifth annual list of the 100 most promising private AI companies.
What they found: The list of 100 was drawn from over 6,000 contenders based on measures including number and type of investors, R&D activity, news sentiment analysis, and competitive landscape. (Disclosure: Landing AI, where Andrew is CEO, is on the list.)
- Just over half of the companies selected provide services such as machine learning operations (MLOps) that feed tech’s appetite for AI. The rest cater to 18 other industries, mostly healthcare, transportation, retail services, and logistics.
- Collectively, they’ve raised $11.7 billion since 2010. The most richly funded entries include Chinese chipmaker Horizon Robotics ($1.6 billion), American autonomous driving company Aurora ($1.16 billion), and Chinese self-driving outfit Momenta ($783 million). More than a dozen are valued at more than $1 billion.
- Many of the companies are still in early stages. Over a third haven’t made it past Series A funding.
- Sixty-four companies on the list are based in the U.S. The UK has eight, and China and Israel have six each.
Whatever happened to . . . : Twenty-one companies from last year’s list made it to this year’s. Three of last year’s cohort had successful IPOs, one went public outside regular investment channels, and two were acquired. All are still in business.
Why it matters: In the midst of massive global economic turmoil, the AI industry continues to prosper. But, while AI’s impacts are global, U.S. companies continue to scoop up most of the rewards.
We’re thinking: Building companies is hard. To quote Theodore Roosevelt, credit should be given to the person “who is actually in the arena, whose face is marred by dust and sweat and blood.” To everyone working on a startup, we wish you success!
A MESSAGE FROM DEEPLEARNING.AI
.png?upscale=true&name=The%20Batch%20(2).png)
We’ve just updated the Deep Learning Specialization with the latest advances! This 2021 release now goes up to Transformer Networks and programming exercises in TF2. Wondering whether to learn deep learning? This is a good time to jump in! Start today
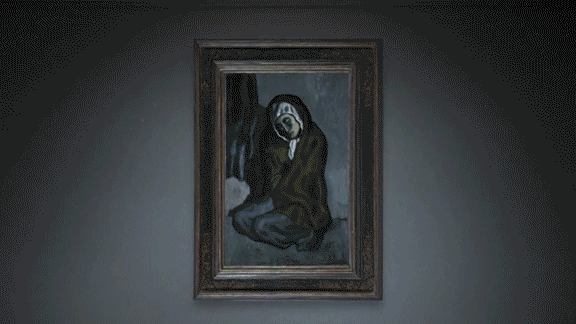
Crouching Beggar, Hidden Painting
Neural networks for image generation don’t just create new art — they can help recreate works that have been lost for ages.
What’s new: Oxia Palus, a UK startup dedicated to resurrecting lost art through AI, combined deep learning and 3D printing to reproduce a painting that had been hidden beneath one of Pablo Picasso’s works.
How it works: In 2018, researchers used an x-ray technique to reveal that Picasso had painted his “Crouching Beggar” on top of another artwork. Art experts believe the underlying composition, which depicts a park in Barcelona, was painted by Picasso’s contemporary Santiago Rusiñol.
- Anthony Bourached and George Cann, the company’s CEO and CTO respectively, manually derived a black-and-white outline of the hidden painting from the x-ray image.
- They divided the outline into built structures, greenery, and sky. For each component, they chose an existing Rusiñol painting and used a style transfer method to map its style to the relevant areas, producing a composite generated image.
- To capture the topography of the artist’s brushstrokes, they mapped the style of a Rusiñol work painted via the impasto technique, in which paint is applied thickly so that color correlates well with height, onto the generated image. They made a grayscale version of the output to produce a heightmap.
- Working with a specialty 3D printing company, they used the generated image and heightmap to produce a facsimile painting, taking extra care not to deform the canvas beneath layers of paint. The process embeds a unique code in each print as a copy-protection measure.
- Oxia Palas plans to sell 100 copies of the resulting print titled “Parc Del Laberint D’horta” ($11,111.11, NFT included).
Behind the news: The team used an earlier version of its style-transfer method to recreate a portrait of a woman hidden beneath Picasso’s “The Old Guitarist.” More recently, it trained a conditional generative adversarial network on 225 paintings made by Leonardo da Vinci and his students to recreate painted-over portions of da Vinci’s “Virgin on the Rocks.”
Why it matters: The false starts and abandoned ideas hidden under later works of art can offer valuable glimpses into a painter’s creative process. This combination of style transfer and 3D printing reveals what might have been.
We’re thinking: It’s fitting that Picasso, who revolutionized art in the 20th century, is providing inspiration for a new, AI-powered avant garde.
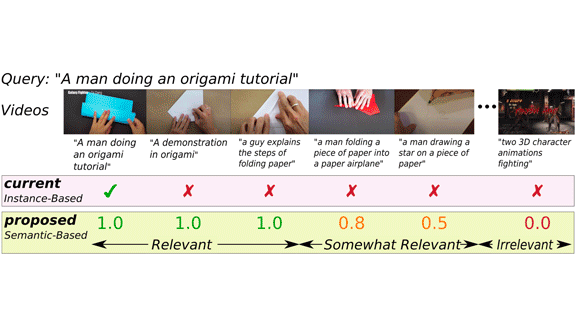
Toward Better Video Search
Video search engines are often evaluated based on how they rank a single video when presented with a brief description that accompanies that video in the test set. But this criterion may not reflect a system's utility in the real world, where numerous videos may be highly relevant to the search terms. New work aims to solve this problem. What’s new: Researchers at the University of Bristol led by Michael Wray propose a new benchmark, Semantic Similarity Video Retrieval (SVR), that evaluates video retrieval systems by their ability to rank many similar videos. They also built a system that performed well on it. Key insight: To evaluate a video retrieval system based on how similar the top-ranked videos are to an input description, the evaluation process needs a ground-truth measure of similarity between descriptions and videos. There isn’t an automatic way to compare a description to a video, but there are several ways to compare a description to other descriptions. The authors assessed the similarity between existing descriptions to approximate ground-truth similarity between descriptions and videos. This enabled them to train their system to rank the similarity of input text to a variety of videos, and to evaluate the quality of its search results. How it works: The authors generated separate representations for captions and videos and honed the similarity of matching descriptions and videos. Given a description, the system learned to rank clips whose video representation best matched that of the input (and vice-versa). They trained and tested it on videos with descriptions from movies, news, how-tos, and other sources.
- The authors calculated similarity between each description and every other description using METEOR. If the similarity between two descriptions exceeded a threshold, they matched the description with the video bearing the other caption.
- They used these matches to train a system that included a GPT-based language model, which generated representations of descriptions, and a combination of convolutional neural networks, which generated representations of videos. A triplet loss encouraged the system to produce similar representations of matched descriptions and videos and dissimilar representations of unmatched ones.
- Given input text (for the purpose of evaluation, an existing description), they ranked the top-matching videos according to the cosine similarity between the representations of the text and the representation of the videos.
Results: The authors measured how well their system ranked each video with respect to every description (and vice-versa) using nDCG. This method rewards high rankings of similar representations (as measured by METEOR) and penalizes high rankings of dissimilar representations. The authors’ system scored 0.840 out of a perfect 1.0. A baseline system that used two vanilla neural networks to create video and description embeddings scored .833. Why it matters: Rather than designing a system to ace a common test, the authors devised a new test that better reflects what users expect from such systems. That approach should lead to more useful systems all around. We’re thinking: The more machine learning improves, the more we need benchmarks that are capable of measuring the latest improvements.
Work With Andrew Ng
Project Manager: DeepLearning.AI seeks a project manager. In this role, you will use your organizational and communication skills to manage course development. You will lead team meetings, track progress, identify and resolve blocks, and address problems as they arise. If project management in a fast-paced environment excites you, this role may be a great fit. Apply here
Subscribe and view previous issues here.
Thoughts, suggestions, feedback? Please send to thebatch@deeplearning.ai. Avoid our newsletter ending up in your spam folder by adding our email address to your contacts list.
Copyright 2021 DeepLearning.AI, 195 Page Mill Road, Suite 115, Palo Alto, CA 94306, United States. All rights reserved.
|