Dear friends,
Last week, I saw a lot of social media discussion about a paper using deep learning to generate artificial comments on news articles. I’m not sure why anyone thinks this is a good idea. At best, it adds noise to the media environment. At worst, it’s a tool for con artists and propagandists.
A few years ago, an acquaintance pulled me aside at a conference to tell me he was building a similar fake comment generator. His project worried me, and I privately discussed it with a few AI colleagues, but none of us knew what to do about it. It was only this year, with the staged release of OpenAI’s GPT-2 language model, that the question went mainstream.
Do we avoid publicizing AI threats to try to slow their spread, as I did after hearing about my acquaintance’s project? Keeping secret the details of biological and nuclear weapon designs has been a major force slowing their proliferation. Alternatively, should we publicize them to encourage defenses, as I’m doing in this letter?
Efforts like the OECD’s Principles on AI, which state that “AI should benefit people and the planet,” give useful high-level guidance. But we need to develop guidelines to ethical behavior in practical situations, along with concrete mechanisms to encourage and empower such behavior.
We should look to other disciplines for inspiration, though these ideas will have to be adapted to AI. For example, in computer security, researchers are expected to report vulnerabilities to software vendors confidentially and give them time to issue a patch. But AI actors are global, so it’s less clear how to report specific AI threats.
Or consider healthcare. Doctors have a duty to care for their patients, and also enjoy legal protections so long as they are working to discharge this duty. In AI, what is the duty of an engineer, and how can we make sure engineers are empowered to act in society’s best interest?
To this day, I don’t know if I did the right thing years ago, when I did not publicize the threat of AI fake commentary. If ethical use of AI is important to you, I hope you will discuss worrisome uses of AI with trusted colleagues so we can help each other find the best path forward. Together, we can think through concrete mechanisms to increase the odds that this powerful technology will reach its highest potential.
Keep learning!
Andrew
News
Tesla Bets on Slim Neural Nets
Elon Musk has promised a fleet of autonomous Tesla taxis by 2020. The company reportedly purchased a computer vision startup to help meet that goal. What’s new: Tesla acquired DeepScale, a Silicon Valley startup that processes computer vision on low-power electronics, according to CNBC. The price was not reported.
- DeepScale, founded in 2015 by two UC Berkeley computer scientists, had raised nearly $19 million prior to Tesla’s purchase.
- The company’s platform, called Carver21, uses a high-efficiency neural network architecture known as SqueezeNet.
- The systems uses three parallel networks to perform object detection, lane identification, and drivable area identification.
- Carver21 imposes a computational budget of 0.6 trillion operations per second. That’s a relatively small demand on Tesla’s custom chipset, which is capable of 36 trillion operations per second.
Behind the news: Tesla’s stock is down 25 percent this year due to manufacturing problems and a drop in demand for electric vehicles. In July, the company lost around 10 percent of its self-driving dev team after Musk expressed displeasure at their inability to adapt its highway-specific autopilot software to urban driving, according to a report in The Information. The recent debut of Tesla’s Smart Summon feature, which enables cars to drive themselves from a parking space to their waiting owner, was marred by reports of accidents. Why it matters: Cars operate within tight constraints on electrical power, and self-driving cars consume lots of power-hungry processing. Tesla is betting that leaner processing will help it reach full autonomy within the power budget of an electric vehicle. Fleets of self-driving taxis would certainly bolster the company’s bottom line. We’re thinking: Low-power processing is just one of many things that will make fully self-driving systems practical. There's widespread skepticism about Tesla's ability to deliver on its promises on time, but every piece will help.
Hidden Findings Revealed
Drugs undergo rigorous experimentation and clinical trials to gain regulatory approval, while dietary supplements get less scrutiny. Even when a drug study reveals an interaction with supplements, the discovery tends to receive little attention. Consequently, information about interactions between drugs and supplements — and between various supplements — is relatively obscure. A new model brings it to light. What’s new: Lucy Lu Wang and collaborators at the Allen Institute created supp.ai, a website that scans medical research for information about such interplay. Users can enter a supplement name to find documented interactions. Key insight: Language describing drug interactions is similar to that describing interactions involving supplements, so an approach that spots drug interactions should work for supplements. How it works: The researchers modified an earlier model that finds drug-to-drug interactions in medical literature to support supplements.
- The authors compiled a list of supplements and drugs in the TRC Natural Medicines database of 1,400 supplements and the Unified Medical Language System’s database of 2 million medical terms and their relationships.
- They used a sentence extraction tool to search abstracts of publications indexed by the Medline database for sentences containing references to multiple supplements.
- They fine-tuned a BERT language model on the Merged-PDDI archive of documents describing drug-to-drug interactions.
- Based on patterns in that archive, the model predicted whether a sentence describes drug-to-drug, supplement-to-drug, or supplement-to-supplement interactions.
Results: Among 22 million abstracts, the system classified 1.1 million sentences describing interactions. To assess accuracy, the authors hand-labeled 400 sentences that contained references to supplements. On this subset, the system was 87 percent accurate in identifying supplement interactions, compared with 92 percent for drug interactions, the state of the art in that task. Why it matters: Most U.S. adults use a dietary supplement, yet their interactions with drugs or one another are virtually unknown. Supp.ai makes it easy for anyone with a web browser to look them up. We’re thinking: The researchers took advantage of the similarity between text discussing drug and supplement interactions to adapt a drug-oriented model for an entirely different, less-scrutinized class of remedies — a clever approach to a difficult problem.
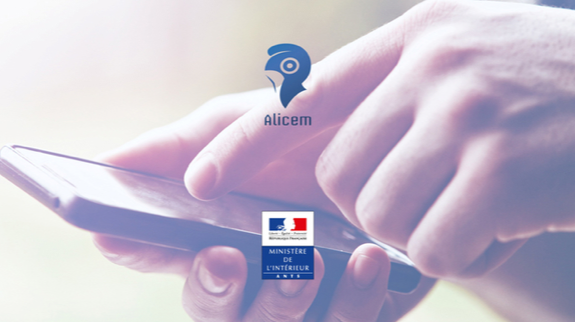
Want Your Pension? Send a Selfie
The French government plans to roll out a national identification service based on face recognition. Critics warn that the new system violates citizens' privacy. What’s new: Beginning in November, President Emmanual Macron’s administration plans to implement a digital ID program based on an Android app. While French citizens aren't required to enroll, the app will be the only digital portal to many government services. How it works: Called Alicem, the app is designed to authenticate the user’s identity for interactions such as filing taxes, applying for pension benefits, and paying utility bills.
- Alicem starts by capturing video of the user’s face from various angles.
- Then it compares the video to the user’s passport photo to determine whether they depict the same individual.
- The app will delete the video selfie once it has completed enrollment, according to France’s Ministry of Interior.
Behind the news: France isn’t the first government to use face recognition in this way. Singapore also offers access to government services via face print. Yes, but: Emilie Seruga-Cau, who heads France’s privacy regulator, says Alicem’s authentication scheme violates the European Union’s General Data Protection Regulation by failing to offer an alternative to face recognition. A privacy group called La Quadrature du Net has sued the government over the issue. France’s Interior Ministry has shown no sign of bowing to such concerns. We’re thinking: Any democratic government aiming to use face recognition for identification must protect its citizens on two fronts. Laws must restrict use of the data to its intended purpose, and due care must be taken to secure the data against hacks.
A MESSAGE FROM DEEPLEARNING.AI
Trying to understand the latest AI research but struggling to understand the math? Build your intuition for foundational deep learning techniques in the Deep Learning Specialization. Enroll now
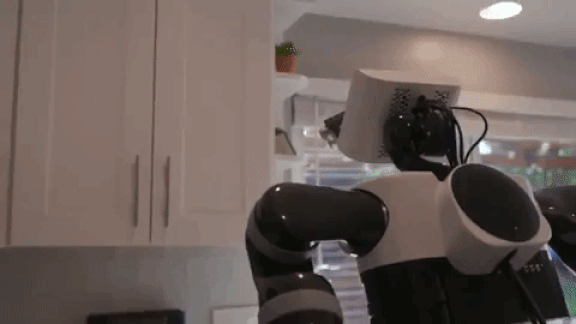
A Robot in Every Kitchen
Every home is different. That makes it difficult for domestic robots to translate skills learned in one household — say, fetching a soda from the fridge — into another. Training in virtual reality, where the robot has access to rich information about three-dimensional objects and spaces, can make it easier for robots to generalize skills to the real world. What’s new: Toyota Research Institute built a household robot that users can train using a virtual reality interface. The robot learns a new behavior based on a single instance of VR guidance. Then it responds to voice commands to carry out the behavior in a variety of real-world environments. How it works: Toyota’s robot is pieced together from off-the-shelf parts, including two cameras provide stereoscopic vision. Classical robotics software controls the machine, while convolutional neural networks learn unique embeddings.
- To teach the robot new tasks, a user wears a VR headset to see through its eyes and drive it via handheld paddles.
- During training, the system maps each pixel to a wealth of information including object class, a vector pointing to the object's center, and other features invariant to view and lighting.
- When the robot carries out a learned action in the real world, it establishes a pixel correspondence between its training and the present scene, and adjusts its behavior accordingly.
Results: The Toyota researchers trained the bot in the virtual environment on three tasks: retrieving a bottle from a refrigerator, removing a cup from a dishwasher, and moving multiple objects to different locations. Then they had the robot perform each task 10 times in two physical homes. They ran the experiments with slight alterations, for instance asking the robot to retrieve a bottle from a higher shelf than the virtual one it was trained on, or doing so with the lights turned off. The robot achieved an 85 percent success rate — though it took an average 20 times longer than a human would. Why it matters: Researchers have given a lot of attention lately to the use of reinforcement learning on robots that are both trained and tested in a simulated environment. Getting such systems to generalize from a simulation to the real world is an important step toward making them useful. We’re thinking: Birth rates have been slowing for decades in Japan, China, the U.S., and much of Europe. The World Health Organization estimates that 22 percent of the world’s population will be over 60 years old by 2050. Who will care for the elderly? Robots may be part of the answer.
Deep Learning Tackles Skin Ailments
Skin conditions are the fourth-largest cause of nonfatal disease worldwide, but access to dermatological care is sparse. A new study shows that a neural network can do front-line diagnostic work. What’s new: Researchers at Google Health, UCSF, MIT, and the Medical University of Graz trained a model to examine patient records and predict the likelihood of 26 common skin diseases. The researchers believe that their system could improve the diagnostic performance of primary-care centers for skin disease. Key insight: The system is designed to mimic the typical diagnostic process in a teledermatology setting. It accepts a patient’s medical history and up to six images, and returns a differential diagnosis, or a ranked list of likely diagnoses. How it works: Yuan Liu and her colleagues collected anonymized patient histories and images from a dermatology service serving 17 sites across two U.S. states. They trained on data collected a few years ago, and they tested on data generated more recently to approximate real-world conditions. The system includes:
- A separate Inception-v4 convolutional neural network for each patient image, all of which used the same weights.
- A module that converts patient metadata into a consistent format via predefined rules. A fully connected layer combines images and metadata to predict the probability of a particular disease or an “other” class.
Results: The model classified diseases more accurately than primary care physicians and nurse practitioners. Allowed three guesses, it was more accurate than dermatologists by 10 percent. The system proved robust to skin color and type and its performance remained consistent across variations. Why it matters: Most previous models consider only a single image and classify a single disease. Inspired by current medical practices, this model uses a variable number of input images, makes use of non-visual patient information as well, and classifies a variety of conditions. The research also shows how to establish model robustness by comparing performance across characteristics like skin color, age, and sex. Yes, but: This study drew data from a limited geographic area. It remains to be seen whether the results generalize to other regions or whether such systems need to be trained or fine-tuned to account for specific geographic areas. We're thinking: Computer vision has been making great progress in dermatology. Still, there are many difficult steps between encouraging results and deployment in clinical settings.
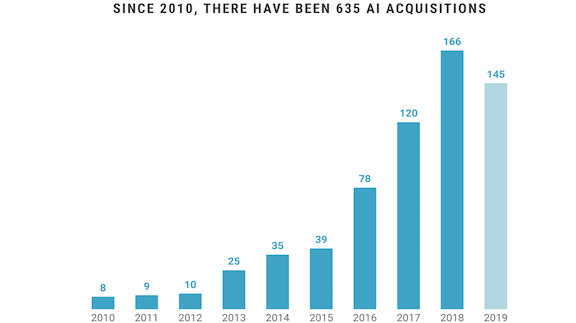
AI Startups in Demand
AI startups are being scooped up at an accelerating pace, many by companies outside the tech sphere. What’s new: A report by CB Insights shows that, as of August, 2019 was on track to surpass last year’s record number of AI startup acquisitions. The annual tally has grown an average of 38 percent every year since 2010. Who’s buying: While tech giants buy more startups on average, non-tech companies account for the overwhelming majority of purchases.
- Apple has the biggest portfolio, having acquired 20 AI startups since 2010, including the companies behind popular features like Siri and FaceID.
- Amazon, Facebook, Google, Microsoft, and Intel are the other notable customers, each having acquired at least seven companies working on computer vision, natural language processing, speech recognition, and the like.
- Most acquisitions by far have been one-off purchases by incumbents outside tech. For instance, John Deere, McDonalds, and Nike snatched up companies that help do things like harvest crops, develop customer relationships, and manage inventory.
What they’re paying: Seven AI acquisitions topped a billion dollars. The most recent happened in April, when pharma giant Roche Holdings closed its $1.9 billion purchase of cancer analytics provider Flatiron Health. The report doesn’t provide annual spending totals. Why it matters: The report makes a strong case that AI’s strategic value is rising steadily throughout the economy. AI is still a tech-giant specialty, but it’s becoming essential in industries well beyond the internet and software. We’re thinking: Exciting startups attract talent, and their work leads to acquisitions that supercharge innovation with bigger budgets and wider reach, drawing still more people into the field. The latest numbers show that this virtuous cycle has staying power — enough, perhaps, to overcome the ongoing shortage of machine learning engineers.
Thoughts, suggestions, feedback? Please send to thebatch@deeplearning.ai. Subscribe here and add our address to your contacts list so our mailings don't end up in the spam folder. You can unsubscribe from this newsletter or update your preferences here.
Copyright 2019 deeplearning.ai, 195 Page Mill Road, Suite 115, Palo Alto, California 94306, United States. All rights reserved.
|