Dear friends,
Last Wednesday, the U.S. Capitol building was overrun by insurrectionists at the moment when members of Congress were certifying the results of a national election. Reading accounts of how close the mob came to where those representatives had sheltered, I believe the legislative branch came closer to falling than many people realize. This event was unprecedented, and its consequences will be playing out for a long time.
U.S. democracy has taken a lot of damage in recent years. Citizens have become polarized. Some politicians have become brazen in their disregard for facts. Voters have been suppressed. The press has been vilified and attacked. Similar things have happened in other countries, and formerly healthy democracies have fallen into populism, authoritarianism, or totalitarianism.
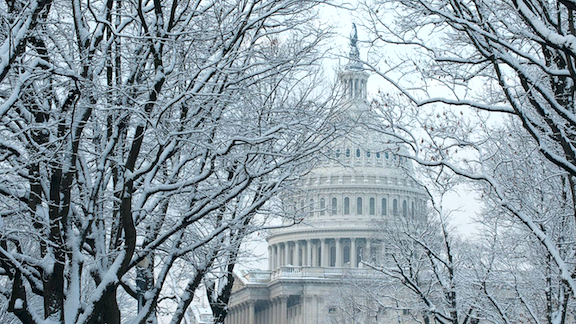
I hope this latest challenge will inspire a renewal of democracy. Organizations that are tested — and that survive the test — end up stronger.
Democracy stands on several pillars, among them:
- Citizens who are informed by truthful perspectives supported by a free press and scientific enquiry
- Institutions that create and enforce laws to make sure that society operates according to rules
- Free and fair elections in which each individual has a vote that counts
The AI community can help strengthen all three.
- As ambiguous information surfaces and is tossed into the grinder of social media, recommendation engines can drive polarization. How can we build recommenders that bring people together rather than driving them apart?
- Decisions to ban polarizing entities — including President Trump — from tech platforms have appeared to be made ad hoc. Instead, they need to be based on rules that are fair and consistently applied. If companies and regulators can develop such rules — which will not be easy — AI can play a significant role in implementing them at scale.
- Digital tools have been used to selectively discourage voting and to gerrymander. On the positive side, they’ve also been used to inform voters and drive turnout. We need to develop new categories of tools and muster the political will to use them o empower all voters.
January 6, 2021, was a nadir for the U.S., and the path ahead will be long and hard. But I believe the country has reached a turning point. I hope the dire events of the past week will renew our appreciation of just how precious sound government is.
Keep learning!
Andrew
News
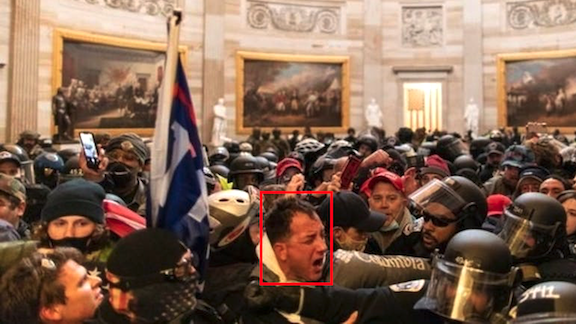
AI Truths, AI Falsehoods
Face recognition is being used to identify people involved in last week’s assault on the U.S. Capitol. It’s also being misused to support their cause. What’s new: Law enforcement agencies and online sleuths are using deep learning to put names to faces in images shot while supporters of U.S. President Trump overran the building in Washington, D.C. to stop certification of his defeat in the recent national election, leaving several people dead and many injured. At the same time, pro-Trump propagandists are making false claims that the technology shows left-wing infiltrators led the attack. What happened: Police arrested few of the perpetrators. In the aftermath, the abundant images have fed AI-powered sleuthing to find those who were allowed to leave the scene.
- University of Toronto researcher John Scott-Railton used face identification and image enhancement to help identify a man who was photographed inside the Senate chamber wearing body armor and carrying zip-tie handcuffs as retired Air Force Colonel Larry Rendall Brock, Jr. Subsequently Brock was arrested.
- Clearview AI, a face recognition company used by thousands of U.S. law enforcement agencies, saw a 26 percent jump in search requests following the attack. At least two police agencies have acknowledged using the service to identify perpetrators.
- Even as face recognition determined that some of the most visible leaders of the assault were Trump supporters, the right-leaning Washington Times erroneously reported that face recognition vendor XRVision had identified individuals leading the assault as left-wing Antifa activists. XRVision called the story “outright false, misleading, and defamatory.”
Deepfakes, too: Falsehoods also circulated regarding deepfake technology. Users of 4chan and social media site Parler wrongly asserted that President Trump’s post-insurrection speech, in which he called the participants “criminals” and “unpatriotic,” was faked by AI. The White House debunked this claim. Why it matters: The Capitol assault, apart from its aim to disrupt the democratic process (and apparently to assassinate officials), highlights that face recognition and deepfakes are two sides of the machine learning coin: One is a powerful tool for uncovering facts, the other a powerful tool for inventing them. While the police are relying on the former capability, propagandists are exploiting both by spreading believable but false claims.
We’re thinking: Paranoia about artificial intelligence once centered on fear that a malicious superintelligence would wreak havoc. It turns out that humans using AI — and lies about AI — to spread disinformation pose a more immediate threat.
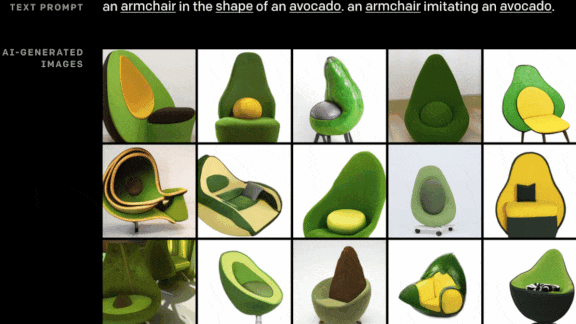
Tell Me a Picture
Two new models show a surprisingly sharp sense of the relationship between words and images. What’s new: OpenAI, the for-profit research lab, announced a pair of models that have produced impressive results in multimodal learning: DALL·E, which generates images in response to written prompts, and Contrastive Language-Image Pretraining (CLIP), a zero-shot image classifier. The company published a paper that describes CLIP in detail; a similar Dall-E paper is forthcoming. How they work: Both models were trained on text-image pairs.
- DALL·E (whose name honors both Salvador Dalí and Pixar’s WALL·E) is a decoder-only transformer model. OpenAI trained it on images with text captions taken from the internet. Given a sequence of tokens that represent a text and/or image, it predicts the next token. Then it predicts the next token given its previous prediction and all previous tokens.
- This allows DALL·E to generate images from a wide range of text prompts and to generate fanciful images that aren’t represented in its training data, such as “an armchair in the shape of an avocado.”
- CLIP uses a text encoder (a modified transformer) and an image encoder (a vision transformer) trained on 400 million image-text pairs drawn from the internet. Using contrastive loss function adopted from ConVIRT, it learned to predict which of nearly 33,000 text snippets would match an image.
- Since CLIP can predict which text best matches an image among any number of texts, it can perform zero-shot classification in any image classification task. At inference, CLIP is given a list of all potential classes in the form of “a photo of a {object}.” Then, fed an image, it returns the most likely class from the list.
Yes, but: Neither model is immune to goofs. Asked to produce a pentagonal clock, for instance, DALL·E rendered some timepieces with six or seven sides. CLIP, meanwhile, has trouble counting objects in an image and differentiating subclasses like car brands or flower species.
Behind the news: The new models build on earlier research at the intersection of words and images. A seminal 2016 paper from the University of Michigan and Max Planck Institute for Informatics showed that GANs could generate images from text embeddings. Other work has resulted in models that render images from text, among them Generative Engine and Text to Image. Judging by the examples OpenAI has published so far, however, DALL·E seems to produce more accurate depictions and to navigate a startling variety of prompts with flair. Why it matters: As OpenAI chief scientist (and former post-doc in Andrew’s lab) Ilya Sutskever recently wrote in The Batch, humans understand concepts not only through words but through visual images. Plus, combining language and vision techniques could overcome computer vision’s need for large, well labeled datasets. We’re thinking: If we ever build a neural network that exhibits a sense of wonder, we’ll call it GOLL·E.
.gif?upscale=true&name=ezgif.com-gif-maker%20(61).gif)
Striding Toward the Minimum
When you’re training a deep learning model, it can take days for an optimization algorithm to minimize the loss function. A new approach could save time. What’s new: Juntang Zhuang and colleagues at Yale, University of Illinois at Urbana-Champaign, and University of Central Florida proposed AdaBelief, a more efficient variation on the popular Adam optimizer. Key insight: The popular optimization methods of stochastic gradient descent (SGD) and Adam sometimes take small steps, requiring more time to reach their destination, when they could take larger ones. Given a small learning rate and a point in a large, steep area of a loss function’s landscape, SGD takes small steps until the slope becomes steeper, while Adam’s steps become smaller as it progresses. In both scenarios, an ideal optimizer would predict that the slope is long and take larger steps. How it works: AdaBelief adjusts its step size depending on the difference between the current gradient and the average of previous gradients.
- Like Adam, AdaBelief moves along a function step by step and calculates an exponential moving average of the gradient, assigning exponentially smaller weights to previous gradients. Also like Adam, at each step, a steeper average gradient generally calls for a larger step size.
- Unlike Adam, AdaBelief treats the weighted average as a prediction of the gradient at the next step. If the difference between the prediction and the actual gradient is small, the function’s steepness probably isn’t changing much, and AdaBelief takes a relatively larger step. Conversely, if the difference is large, the landscape is changing, and AdaBelief decreases the step size.
Results: The authors provide videos showing that, in experiments on functions with known minimums, AdaBelief was faster than both Adam and SGD with momentum (as shown above). To demonstrate their method’s accuracy, they compared AdaBelief to SGD, Adam, and other adaptive optimizers on tasks including image classification, image generation, and language modeling. AdaBelief basically matched SGD’s accuracy and exceeded that of all other adaptive optimizers. For instance, on ImageNet, AdaBelief increased a ResNet18’s highest top-1 accuracy, or accuracy of its best prediction, to 70.08 percent, on par with SGD’s 70.23 percent and 2 percent better than the best adaptive optimizers. Why it matters: Faster optimization means faster training, and that means more time to experiment with different models. We’re thinking: The authors’ video demonstrations suggest that AdaBelief could be a valuable alternative to Adam. However, they don’t supply any numbers that would make for a precise speed comparison. We look forward to the authors of the Deep Learning Optimizer Benchmark Suite, who have evaluated over a dozen optimizers in various tasks, running AdaBelief through its paces.
A MESSAGE FROM DEEPLEARNING.AI
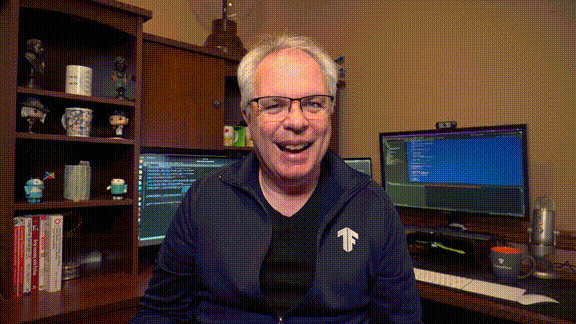
“Generative Deep Learning with TensorFlow,” Course 4 of our TensorFlow: Advanced Techniques Specialization, is now available on Coursera. Enroll now
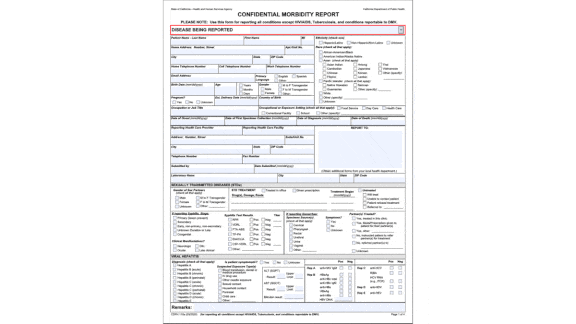
The Fax About Tracking Covid
A pair of neural networks is helping to prioritize Covid-19 cases for contact tracing. What’s new: The public health department of California’s Contra Costa County is using deep learning to sort Covid-19 cases reported via the pre-internet technology known as fax. How it works: Hospitals and medical labs document cases of coronavirus infection using hand-written forms. Many transmit the documents to public health officials over telephone landlines. Stanford University researchers developed Covid Fast Fax to evaluate them so that public health workers, who still manually review each case, can spot the most critical ones. The system comprises two convolutional neural networks.
- One model culls Covid-19 reports from other incoming faxes. The researchers trained it using 25,000 copies of the five forms used most frequently by area hospitals. They augmented the dataset by adding blurs, streaks, and other distortions commonly seen in fax transmissions.
- The second model determines which reports are most urgent. It ranks the severity of each case by reading checkboxes that indicate a patient’s symptoms, gender, isolation status, and other details. To train it, the researchers wrote 130 fake reports, transmitted them by fax, and augmented them by flipping, blurring, and adding noise.
- The researchers evaluated their system on 1,224 faxes received over a two week period. The system was able to read 88 percent of the documents. Of these, it detected Covid-19 reports with 91 percent recall, a measurement for accuracy that docks the model for mislabeling high-priority cases.
Behind the news: The use of fax in health care persists despite billions of dollars to promote digital health records. Digital systems face roadblocks, as many professionals find them difficult to use, and for-profit hospitals aren’t always eager to make it easy for patients to share their information with competitors.
Why it matters: According to a 2019 survey, 89 percent of U.S. health organizations still rely on fax to transmit medical information. Anything that accelerates the processing of that information is a plus — especially during a pandemic. We’re thinking: It’s 2021, and hospitals are still relying on fax to make critical decisions? AI can help hospitals cope with outmoded communications technology, but it’s no substitute for updating U.S. health care infrastructure.
.gif?upscale=true&name=ezgif.com-gif-maker%20(69).gif)
It’s a Small World Model After All
World models, which learn a compressed representation of a dynamic environment like, say, a video game, have delivered top results in reinforcement learning. A new method makes them much smaller. What’s new: Jan Robine and colleagues at Heinrich Heine University Düsseldorf present Discrete Latent Space World Models. Their approach matches the performance of the state of the art in six Atari games, SimPLe, with far fewer parameters. Key insight: Researchers have devoted significant effort to making reinforcement learning algorithms efficient, but they’ve given less attention to making models themselves efficient. Using high-performance architectures for the various components of a world model ought to improve the entire system — in this case, by reducing its size. How it works: Following the typical world models approach, the authors trained separate neural networks to generate a representation of the environment (the representation model), predict how actions would affect the environment (the dynamics model), and choose the action that will bring the greatest reward (the policy model).
- For the representation model, the authors used a vector quantized variational autoencoder (VQ-VAE) that’s smaller than the autoencoder in SimPLe. The VQ-VAE takes as input the pixels of a game’s most recent four frames. Its encoder generates a 6x6 matrix of indices, each pointing to a vector in an embedding that represents the environment. (After training, the decoder is no longer needed.)
- For the dynamics model, they used a convolutional LSTM that takes as input the encoder’s output. They trained it to predict the reward and features of the next four frames. Errors backpropagate through to the embedding, so eventually it encodes information about predicted rewards and states. (After training, the dynamics model is no longer needed.)
- For the policy model, they used a small convolutional neural network that also receives the encoder’s output. They trained it to choose an action using proximal policy optimization.
- To train the system, the authors used the same iterative procedure as SimPLe. They let the system interact with the environment, trained the representation and dynamics models, and then trained the policy network; then they repeated the cycle.
Results: The authors compared their method to SimPLe in six Atari games. SimPLe uses 74 million parameters, while their method uses 12 million during training and 3 million during inference. Nonetheless, their method’s mean scores over five training runs beat SimPLe in five out of six games when given 100,000 observations. Yes, but: Although the authors’ method beat SimPLe on average, SimPLe racked up higher scores in four out of six games. Why it matters: Smaller models consume less energy, require less memory, and execute faster than larger ones, enabling machine learning engineers to perform more experiments in less time. We’re thinking: World models are young enough that something as simple as changing the components used can make a big difference. This suggests that plenty of opportunity remains to improve existing models.
A MESSAGE FROM WORKERA
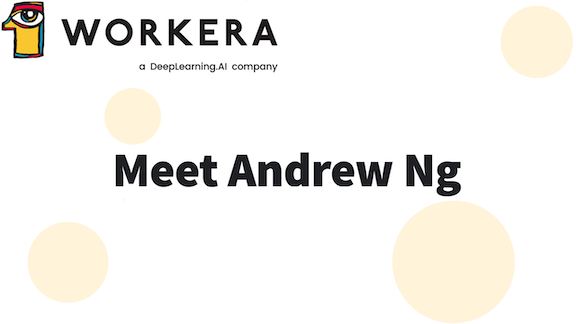
Complete a Workera assessment between January 13, 2021, and February 13, 2021, to qualify for a virtual coffee chat with Andrew Ng! To celebrate the release of Workera’s new Data Literacy Assessment, four members of the Workera community will be selected. Learn more
Machine Learning Instructor: FourthBrain.AI, an AI Fund portfolio company, is looking for an instructor to join its early stage AI training startup. The Instructor will work closely with the curriculum development director to manage students in a part-time ML training program. Apply here
Senior Software Engineer-Front End: Workera, a DeepLearning.AI affiliate, is looking for software engineers experienced in front-end development. In this role, you will build the world’s leading assessment technology to help members of the AI community evaluate their skills and know where they stand. Apply here
Assessment Developer Python/SQL/R/TensorFlow (contractor): Workera, a DeepLearning.AI affiliate, is looking for assessment developers with deep Python/SQL/R/TensorFlow expertise to help build the world’s best assessment of AI skills. You’ll join a team of experts in data science and education implementing quantitative and qualitative methods to improve our online assessment. Apply here
Enterprise Sales Development Representative: Workera, a DeepLearning.AI affiliate, is looking for an enterprise sales rep to drive new business opportunities by qualifying and nurturing leads for the global enterprise sales team in support of our rapidly growing business. Apply here
Subscribe and view previous issues here.
Thoughts, suggestions, feedback? Please send to thebatch@deeplearning.ai. Avoid our newsletter ending up in your spam folder by adding our email address to your contacts list.
Copyright 2021 DeepLearning.AI, 195 Page Mill Road, Suite 115, Palo Alto, CA 94306, United States. All rights reserved.
|