Dear friends,
When it comes to artificial intelligence, one of the biggest mistakes large companies make is thinking tactically rather than strategically.
What's the difference? Some taxi companies thought they had the internet revolution "covered" because they built a website. Then ride-sharing startups disrupted the industry with internet-connected mobile apps that transformed the ride-hailing experience.
Similarly, some companies’ response to AI starts and ends with tactically building a few small projects. But the strategic question is: How will AI transform your industry's core business, and how will that change what it takes for your company to thrive?
I spoke about this at TechCrunch’s conference on Thursday, and Fortune published a nice summary of my remarks. It's not too late for traditional companies to develop a strategic plan to take advantage of AI. The technology is only beginning to find its way into applications outside of software development. But for many companies, it will be critical to act quickly.
AI transformation should start with concrete projects, but it cannot end there. I hope more CEOs learn about AI and think strategically about it.
Keep learning!
Andrew
deeplearning.ai Exclusive
The Tech Accelerator
Omoju Miller’s journey from comp-sci undergrad to GitHub was anything but straightforward. Learn about her day-to-day as a senior machine learning engineer in the latest installment of our Working AI series. Read more
News
Can AI Wage War? Should It?
The U.S. military is developing a new generation of automated weaponry. Some people are calling for automated generals as well.
What happened: A pair of defense experts argue in War on the Rocks, an online publication covering national security, that the Pentagon should replace the human chain of command over nuclear defense with machines. The time available to respond to incoming warheads has dwindled from hours during the Cold War to roughly 6 minutes today. The change makes automated command-and-control a necessity, they say. Their analysis added urgency to feature stories on military AI published last week in The Atlantic and The Economist. Behind the news: The Department of Defense’s 2019 budget calls for nearly $1 billion in AI spending. Almost one-third of the money will fund the Joint Artificial Intelligence Center dedicated to establishing and scaling up AI throughout the military. The remainder of the department's AI budget will support initiatives led by individual branches. Among those efforts:
- The Air Force is developing SkyBorg, an AI copilot for F-16s to help with navigation, radar awareness, and target recognition. The system will also pilot Valkyrie drones (pictured above) to serve as autonomous wingmen.
- The Marine Corps is building self-driving assault boats to deliver troops to a beach, then support the landing via autonomous .50 caliber machine guns.
- The Navy is testing Sea Hunter, an autonomous ship intended eventually to destroy enemy submarines without human intervention.
The controversy: The debate over automated warfare follows trench lines similar to those of the earlier (and ongoing) argument over nuclear weapons.
- Pro-AI military experts fear that developments like hypersonic missiles — capable of traveling up to 20 times the speed of sound — could decapitate the U.S. military before it has a chance to react. They argue that AI-driven warfare is imperative, if only for effective defenses.
- But critics such as The Bulletin of the Atomic Scientists warn that hackers, faulty code, or wayward machines could handicap AI-driven weapons. Moreover, non-nuclear autonomous weaponry could violate ethical and legal codes in the Geneva Conventions.
We're thinking: Autonomous weapons are terrifying enough. Autonomous nuclear weapons verge on the unthinkable. We strongly support the United Nations' effort to establish a ban on autonomous weapons as a complement to nuclear disarmament. That said, AI has potential nonlethal uses like mine removal and search and rescue. It will take vigorous, well informed argument to arrive at military uses of AI that improve conditions for humanity as a whole. It’s critical that the AI community play an active role in the discussion.
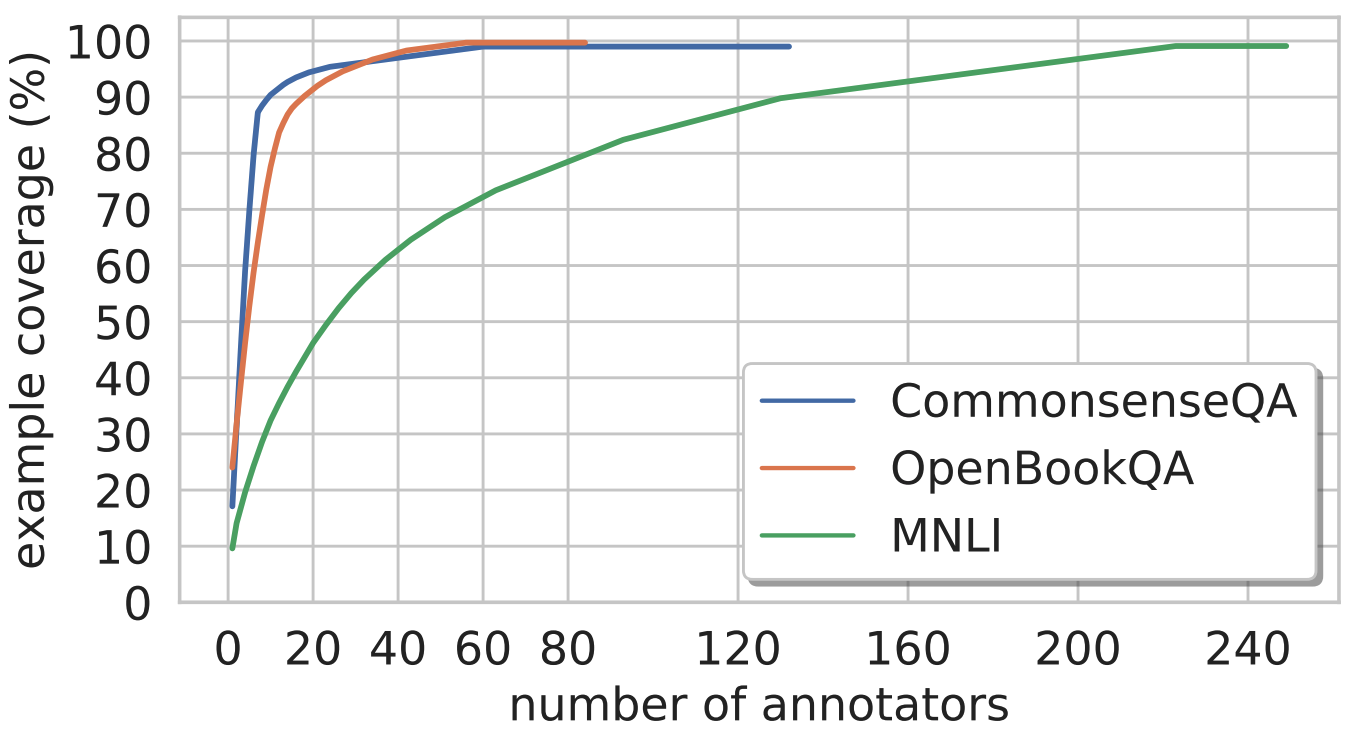
What Language Models Know
Watson set a high bar for language understanding in 2011, when it famously whipped human competitors in the televised trivia game show Jeopardy! IBM’s special-purpose AI required around $1 billion and a squadron of engineers. New research suggests that today’s best language models can accomplish similar tasks right off the shelf.
What's new: Researchers at Facebook AI Research and University College London pitted top-shelf language models against task-specific networks in a Jeopardy!-like challenge they call Language Model Analysis (LAMA). Their LAMA data set provides a large corpus of sentences, each missing a key fact. Key Insight: The latest language models are pretrained to address a variety of downstream tasks. In learning language representations, they retain knowledge that can be used to complete statements lacking key words. How it works: LAMA builds its incomplete sentences based on facts drawn from Google-RE (facts from Wikipedia), T-REx (facts aligned with Wikipedia text), ConceptNet (a semantic network), and SQuAD (questions and answers).
- LAMA requires models to fill in a missing subject or object. For example, “The theory of relativity was developed by ___.”
- The researchers evaluated off-the-shelf versions of BERT, ELMo, and Transformer-XL without further training.
Results: BERT-Large filled in the blanks most accurately overall, and it was best at completing statements based on Google-RE and ConceptNet. It proved only half as accurate as task-specific models on LAMA’s SQuAD portion, which contains more complicated sentences. Similarly, BERT’s performance suffers when T-REx facts contain multiple subjects or blanks. Why it matters: The Allen institute last week reported using BERT to score better than 90 percent on the multiple-choice questions in the New York Regents science test for the eighth grade. That system included additional task-specific models and retrieved external information to complete tasks. This research suggests that BERT as-is would score well on the Regents test.
Takeaway: Large, pretrained language models can glean and recall nearly as much information — from some data sets, at least — as specially designed question answering models. This knowledge can allow them to accomplish various language tasks, including fill-in-the-blank, without special preparation.
Chimp Recognition
AI is capable of picking faces out of the crowd — even if that crowd is squabbling over bananas in a jungle.
What's new: Researchers at the University of Oxford developed a face recognition app that identifies individual chimpanzees in footage shot in the wilds of Guinea. The work could give wildlife conservation efforts a powerful new tool.
How it works: The group adapted the VGG-M convolutional neural network architecture. They trained the model on roughly 50 hours of footage representing 23 individuals over 14 years.
- The model identified apes as they aged.
- It was able to recognize individuals regardless of low light, poor image quality, and facing away from the camera.
- The researchers pitted their model against a human trained to recognize chimps. The human sorted 42 percent of the images correctly. The model's accuracy was 84 percent.
Behind the news: Zoologists have embraced image recognition for conservation efforts. The technology is counting giraffes in Africa and tracking wolverines in the Pacific Northwest. An innovative application called WildBook that trawls YouTube for wildlife videos has been used to catalog whale shark migrations.
Why it matters: Chimpanzees, like humans, are highly social animals. The ability to track individuals enabled the researchers to map the tribe’s structure. The model generalized well to other primate species in preliminary tests. The researchers suggest that their approach could be used with other animals where a sufficient video record exists.
We’re thinking: Applications like this could help cash-strapped conservation efforts to focus on translating data into action, and reduce the need for invasive, labor-intensive methods like tagging animals with RFID.
A MESSAGE FROM DEEPLEARNING.AI
How can you tell when your neural network is overfitting? Learn how to spot avoidable bias in the Deep Learning Specialization. Enroll now
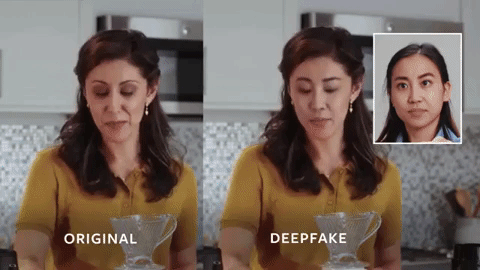
Facing Down Deepfakes
Deepfakes threaten to undermine law and order, perhaps democracy itself. A coalition of tech companies, nonprofits, and academics joined forces to counter potential adverse impacts.
What’s new: The Deepfake Detection Challenge aims to provide a data set of custom-built deepfakes. Funded by a $10 million grant from Facebook, it also promises a prize for developing tools that spot computer-generated pictures. The details: Facebook is producing videos with actors who have consented to having their features altered by deepfake technology.
- A working session at the International Conference on Computer Vision in October will perform quality control.
- Facebook plans to offer access on a limited basis, with full release to follow at the NeurIPS conference in December.
- A competition to identify deepfakes in the dataset will run until spring 2020, with the winner to be awarded an unspecified prize.
- Other partners include Cornell Tech, Microsoft, MIT, the Partnership on AI, UC Berkeley, University at Albany-SUNY, University of Maryland College Park, University of Oxford, and WITNESS.
Behind the news: Activists goaded Facebook to action in June, when they released a synthesized video of Mark Zuckerberg rhapsodizing over his control of billions of peoples’ data. Why it matters: Deepfakes often are portrayed as a potential vector for political disinformation. But, as Vice and Wired point out, the clear and present danger is harassment of individuals, particularly women, activists, and journalists.
We're thinking: The fact that deepfakes are created by adversaries means the data set — and resulting filters — will need to evolve as the fakers adapt to detection algorithms.
Can you spot fakes? Test your personal deepfake radar via this online guessing game.
Leveling the Playing Field
Deep reinforcement learning has given machines apparent hegemony in vintage Atari games, but their scores have been hard to compare — with one another or with human performance — because there are no rules governing what machines can and can’t do to win. Researchers aim to change that.
What’s new: Most AI research demonstrating superhuman performance in Atari games applies widely varying limits on gameplay, such as how frequently buttons can be pressed. Researchers from MINES ParisTech and Valeo offer a standardized setup: Standardized Atari Benchmark for Reinforcement Learning (Saber). They use it to achieve a new state of the art in around 60 games from Pong to Montezuma’s Revenge. Key Insight: Marin Toromanoff, Emilie Wirbel, and Fabien Moutarde noticed that the reported human world-record scores average 1,000 times higher than the “expert human player” scores given in the first major deep reinforcement learning paper published in late 2013. Analyzing the settings used in deep learning publications since, the team pinpointed seven potential causes for reported variations in performance.
How it works: The authors propose a set of guidelines designed to match human capabilities. Their benchmark includes a new metric for evaluating models, since the previous human benchmark misrepresents human capabilities.
- Saber removes limitations on gaming time — it takes time for human players to rack up a world record! — rather than the few minutes many researchers allow.
- The benchmark specifies that models can receive only the game screen as input, no further information allowed. For example, they must be able to use all buttons even if some don’t function.
- The benchmark ranks models on a normalized scale in which 0 represents a score obtained by pressing buttons randomly and 1 is the human world record.
Results: The researchers tested a state-of-the-art model, Rainbow-IQN, and achieved an average of only 31% of the best human scores. The model achieved superhuman scores in four of 58 games.
Why it matters: Training reinforcement learning models is so laborious that researchers often don’t bother to reproduce previous results to see how their own stack up. Saber finally provides a consistent basis for comparison. We're thinking: Deep reinforcement learning research is exciting, but a lack of standardized benchmarks has kept the state of the art in a state of ambiguity. Saber signals a new and promising maturity.
.gif?upscale=true&name=ezgif.com-gif-maker%20(1).gif)
Automation's Frontier: Fast Food
Quick-service restaurants are experiencing record-high employee turnover, while labor advocates are pushing for higher wages. Some experts say these forces are propelling the fast food industry toward full automation.
Who’s already automating: The move to put fast food under machine control is already in high gear:
- McDonalds announced on Tuesday its acquisition of Apprente, a company that develops voice-driven conversational agents. The 34-year-old fast-food pioneer has tested automated ordering kiosks since 2003 and recently allocated $1 billion to upgrade the technology.
- In China, Yum! Brands, owner of KFC, Taco Bell, and Pizza Hut, says 50 percent of transactions take place via app or kiosk.
- Zume Pizza of California uses robots to form dough, spread sauce (pictured above), and bake the resulting pies. Humans place toppings.
- At Spyce in Boston, customers order and pay by kiosk, and a machine mixes their grain-based meals. Human prep cooks par-bake rice, chop veggies, and reduce sauces.
Behind the news: Humans are opting out for the quick-service business. In July, the CEO of Panera Bread told CNBC’s @Work conference that his company experienced nearly 100 percent annual employee turnover — and this number was low for the industry. Turnover in the Accommodations and Restaurants category (which includes traditional restaurants as well as hotels) has climbed nearly 15 percent over the last decade, according to the U.S. Bureau of Labor Statistics.
Why it matters: Fast food is shaping up to be a leading edge of an automation wave that could be squeezing lower-skilled, lower-wage employees out of the economy. A 2017 report by the National Council on Compensation Insurance found that, while automation historically replaces human labor, the jobs that remain tend to be higher skilled and better compensated.
We’re thinking: Apps and kiosks are clearly capable of replacing fast-food customer service. Back-of-the-house work like assembling burritos and stacking sandwiches requires more dexterity. While those positions likely persist longer, it may be cold comfort to find yourself automated out of a job five years from now rather than one.
Thoughts, suggestions, feedback? Please send to thebatch@deeplearning.ai. Subscribe here and add our address to your contacts list so our mailings don't end up in the spam folder. You can unsubscribe from this newsletter or update your preferences here.
Copyright 2019 deeplearning.ai, 195 Page Mill Road, Suite 115, Palo Alto, California 94306, United States. All rights reserved.
|