Dear friends,
Like many people in the AI community, I am saddened by the sudden departure from Google of ethical AI researcher Timnit Gebru. Timnit is a tireless champion of diversity and fairness in AI. Her work, for example highlighting bias in face recognition systems, has been a productive influence on many researchers and companies. At the same time, my friend Jeff Dean built Google AI into a world-class engineering organization. I’ve seen him speak up for diversity when no one else in the room was doing so.
Having not yet spoken to either of them, I hesitate to offer my opinion on the matter at this time. But the situation highlights a larger problem in the AI community: lack of a shared set of values (such as fairness, diversity, and transparency) and norms (such as what to do when there’s a problem).
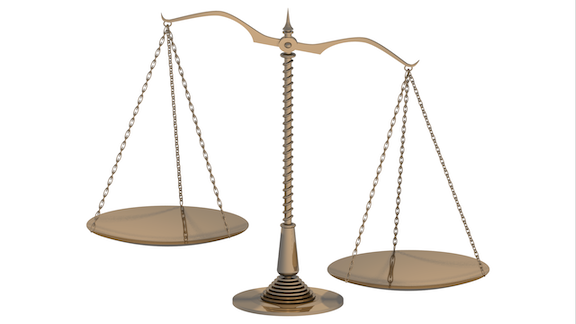
In academia, all scholars place high value on the pursuit and dissemination of knowledge. In medicine, all doctors recognize that the wellbeing of patients is their primary duty. We need that kind universal commitment in AI.
We’re building technology that affects billions of people without a coherent set of guiding principles. Many companies and think tanks have published their own codes of ethics, and these statements are important — but they are far from sufficient. We need a set of values and norms that are shared across our entire community and transcend any one company. That way, we can collectively hold individuals, companies, and perhaps even governments accountable to them and operate for the common good even when we disagree.
How can we bring the AI community together around shared values and norms? I encourage you to spend time with your teams, collaborators, and peers to discuss this difficult question. It’s past time to lay the foundation for a set of values and norms that all AI practitioners will proudly stand up for.
Keep learning!
Andrew
News
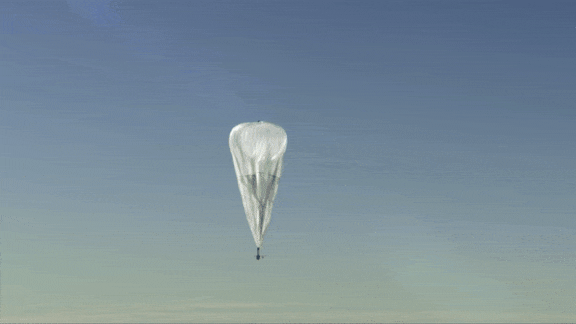
How to Drive a Balloon
Helium balloons that beam internet service to hard-to-serve areas are using AI to navigate amid high-altitude winds.
What’s new: Loon, the Alphabet division that provides wireless internet via polyethylene blimps, used reinforcement learning to develop an autonomous control system that keeps the vehicles closer to their targets while consuming less energy than its hand-coded predecessor. The new algorithm controls Loon’s fleet over Kenya, where the company launched its first commercial service in July.
How it works: Balloons navigate by ascending or descending to catch winds that push them in the direction desired. Loon used QR-DQN, a distributional reinforcement learning algorithm, to train a feed-forward network to determine when the balloon should ascend, descend, or stay put.
- Working with Google AI’s Montreal team, Loon researchers modified a weather dataset from the European Center for Medium-Range Weather Forecasts to generate a large number of wind scenarios. They modeled the physics of balloon flight within these synthesized wind fields to build simulations used to train and evaluate the model.
- In training, the model received the maximum reward when the balloon was within 50 kilometers of its base station, the range at which it reliably sends and receives signals. The reward halved with every 100 kilometers the balloon strayed.
- In use, instruments on board feed the model wind readings from the balloon’s current location and wake. It estimates wind conditions at nearby locations using a Gaussian process that analyzes weather readings from nearby balloons and forecasts from the European Center for Medium-Range Weather Forecasts. A pump inflates or deflates the balloon accordingly.
- In real world tests against the earlier flight control system, the new algorithm stayed on target 7 percent more often while cutting energy consumption by 4 watts day.
Behind the news: Loon began within Alphabet’s experimental X division in the early 2010s and became a for-profit subsidiary in 2018. The company provided emergency internet access to Puerto Rico after hurricane Maria in 2017, and to Peru following a massive earthquake in 2019. A single balloon can serve several thousand individuals spread over 80 square kilometers.
Why it matters: Billions of people, including two-thirds of all school-age children, don’t have access to the internet. In the Covid era, with students and workers alike staying home, the digital divide is more acute than ever. Cutting the cost of service to remote areas could bring many of those people into the information economy. We’re thinking: In Kenya, where Loon’s first balloons are flying, better connections could boost the growing community of AI engineers. To learn more about Kenya’s AI scene, check out our Working AI profile of data scientist and DeepLearning.AI ambassador Kennedy Kamande Wangari.
.gif?upscale=true&name=ezgif.com-gif-maker%20(42).gif)
The Measure of a Muppet
The latest pretrained language models have shown a remarkable ability to learn facts. A new study drills down on issues of scale, showing that such models might learn the approximate weight of a dog or cost of an apple, at least to the right order of magnitude. What’s new: Xikun Zhang and Deepak Ramachandran with colleagues at Stanford, Google, AI2 Israel, Bar Ilan University, and University of Pennsylvania probed whether word embeddings produced by pretrained models encode knowledge of objects’ mass, length, or price. Key insight: Pretrained features that represent words may or may not capture scale-bound attributes. To find out, the authors built simple linear models that took the pretrained embeddings as a starting point and trained them on a dataset that explicitly associates words with such attributes. If the models learned to estimate such attributes, they reasoned, then the pretrained embeddings did, indeed, represent them. How it works: The authors analyzed features generated by ELMo and BERT, whose embeddings vary depending on context, as well as the earlier word2vec, a fixed set of embeddings. They also tested features generated by their own model, NumBERT, which is identical to BERT except that numerals in its pretraining data were replaced by the same numbers in scientific notation.
- The researchers built two linear models that accepted embeddings from each language model. One linear model used regression to produce a median estimate of mass, length, or price. The other produced a distribution of probabilities among 12 orders of magnitude.
- Freezing the language models’ weights, the researchers trained the linear models on the Distribution over Quantities (DoQ) dataset, which contains nouns and distributions of their masses, lengths, and prices.
- They fed the language models sentences like “The dog is heavy” or “The ring is expensive,” and passed the embeddings of the key word (here, “dog” or “ring”) to the linear models to produce an estimate or distribution.
Results: The linear models matched the DoQ measures with greater-than-random accuracy. Those that used embeddings from ELMo, BERT, and NumBERT produced better performance than those that used word2vec. To evaluate whether the linear models generalized beyond DoQ, the authors tested them on comparing sizes and weights between pairs of objects. The regression model that used NumBERT embeddings achieved accuracy of 0.76, outperforming BERT (0.71), ELMo (0.72), and word2vec (0.74). The classification model that used NumBERT embeddings likewise outperformed the others but achieved lower accuracy. Why it matters: The latest language models have come under fire for being less smart than their accomplishments might suggest. But how much less smart? Studies like this help quantify the deficits so we can work toward improving them. We’re thinking: Language models also need to understand scale distinctions based on modifying words such as the difference between “watch” and “gold watch,” or between “Yoda” and “Baby Yoda.”
Prosperity of the Commons
A new consortium of companies, schools, and research labs is building open tools for next-generation machine learning.
What’s new: MLCommons aims to foster innovation in machine learning by developing new benchmarks, datasets, and best practices. Its founding board includes representatives of Alibaba, Facebook, Google, Intel, and DeepLearning.AI’s sister company Landing AI. Fresh resources: The group kicked off by releasing two products:
- People’s Speech contains 87,000 hours of spoken-word examples in 59 languages (mostly English). It includes audio from the internet, audiobooks, and about 5,000 hours of text generated by GPT-3 and spoken by a voice synthesizer.
- MLCube is an interface for sharing models, including data and parameters, via container systems like Docker. Models can run locally or in the cloud.
Behind the news: MLCommons grew out of the development of MLPerf, a benchmark for measuring hardware performance on machine learning tasks. MLCommons will continue to steward MLPerf. Why it matters: Publicly available datasets and benchmarks have spurred much of AI’s recent progress. Producing such resources is expensive, and doing it well requires expertise from several subdisciplines of AI. MLCommons brings together more than 50 organizations to keep the community fueled with the tools necessary to continue innovating. We’re thinking: Datasets from the Linguistic Data Consortium and others have been a boon for speech recognition research in academia, but academic researchers still lack datasets on the scale used by big tech companies. Access to 87,000 hours of speech will help these groups to develop cutting-edge speech systems.
A MESSAGE FROM DEEPLEARNING.AI
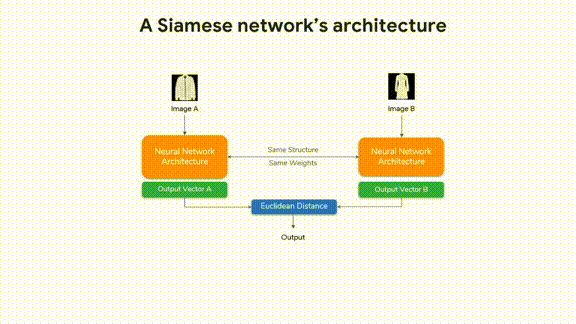
“Advanced Computer Vision with TensorFlow,” Course 3 in our TensorFlow: Advanced Techniques Specialization, is now available on Coursera! Enroll now
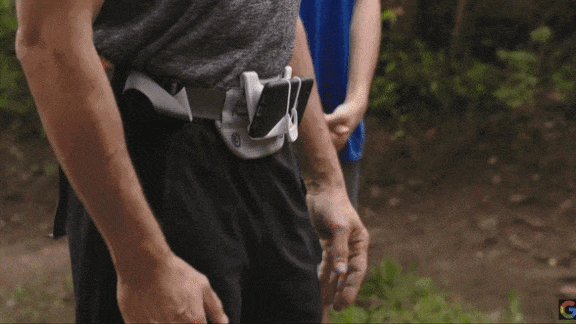
Seeing Eye AI
A computer vision system is helping to keep runners with impaired vision on track. What’s new: A prototype smartphone app developed by Google translates camera images into audio signals. Guidelines produces sounds that indicate deviations from lines painted on a path, enabling people to correct their course without using their eyes, according to VentureBeat. How it works: Users strap an Android device to their belly and listen through bone-conducting headphones that provide both auditory and haptic feedback but don’t block street sounds. All processing is performed on the device and requires no Internet connection.
- The app uses a segmentation model trained on real and synthetic data to identify yellow lines painted on the asphalt, and to ignore white ones. A separate model determines where runners are in relation to the line.
- If the runner drifts off-course to the left or right, a synthesized tone played in the corresponding ear nudges the runner back to center. Different audio frequencies warn runners of upcoming turns and signal them to stop if the system loses sight of the line.
- Guidelines currently works in three New York locations with specially painted road markings and requires a sighted facilitator. The team is working to paint lines in other parks, schools, and public spaces. It aims to collect data from a wider variety of users, locations, weather conditions, road conditions and so on to make the system more robust to a range of conditions.
Behind the news: Other AI-powered accessibility apps are also helping people with sensory impairments live more independently.
- Lookout, also from Google, uses object recognition to help visually impaired people count money and identify packaged foods by their barcodes.
- Microsoft has helped develop apps that assist visually impaired people in maintaining social distance and taking written exams.
- Voiceitt uses speech recognition to help people who have difficulty forming words communicate more clearly.
Why it matters: Apps like this could bring more independence to the hundreds of millions of people worldwide who have a serious visual impairment. And they might help people who can’t see get around town for activities other than exercise. We’re thinking: Some of Andrew’s early efforts in deep learning were inspired by the work of BrainPort Technologies, which showed that you can take an image, map it to a pattern of pressures on a blind person’s tongue, and thereby help them “see” with their tongue. This raises an intriguing question: Is there single learning algorithm that, depending only on the input data it receives, can learn to process either vision or touch?
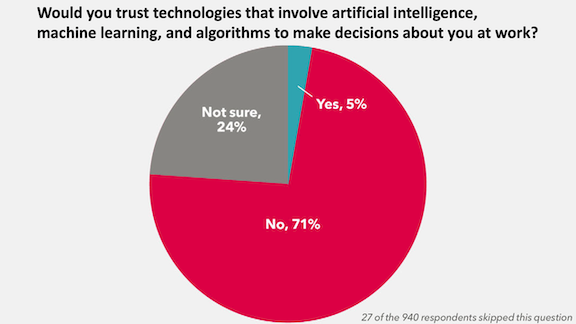
Labor Pushes Back
Labor unions aim to give workers more protection against the automated systems that increasingly rule the workplace. What’s new: The Trades Union Congress (TUC), a federation of unions in England and Wales that represents over 5.5. million workers, launched a task force to lobby for collective bargaining, increased transparency, and legal protections related to AI in the workplace. Challenges of automation: The move comes after the TUC produced a report that found widespread unease among British workers over automated systems that perform management tasks like scheduling shifts, analyzing performance, and determining layoffs.
- Sixty-percent of workers surveyed worried that such tools could lead to unfair treatment.
- Fifty-six percent said automated tools for monitoring the workplace eroded their trust in management.
- Less than one-third said their employers consult with them when new technologies were introduced to the workplace.
- Early next year, the task force plans to publish a report on how to ensure that workplace AI takes workers' needs into account.
Why it matters: Earlier this year, a study found that many companies hesitate to deploy AI models for fear of public and legal backlash. Bringing workers into the process of deciding whether, when, and how to deploy them in the workplace could help overcome the fear and distrust — thought it could also slow AI adoption. We’re thinking: AI is helping companies become more efficient. It also puts more power in the hands of employers by enabling them to manage workers in ways that were not possible before. We welcome efforts to ensure fair treatment of employees.
Work With Andrew Ng
Senior Software Engineer-Front End: Workera, a DeepLearning.AI affiliate, is looking for software engineers experienced in front-end development. In this role, you will build the world’s leading assessment technology to help members of the AI community evaluate their skills and know where they stand. Apply here
Assessment Developer Python/SQL/R/TensorFlow (contractor): Workera, a DeepLearning.AI affiliate, is looking for assessment developers with deep Python/SQL/R/TensorFlow expertise to help build the world’s best assessment of AI skills. You’ll join a team of experts in data science and education implementing quantitative and qualitative methods to improve our online assessment. Apply here
Enterprise Sales Development Representative: Workera, a DeepLearning.AI affiliate, is looking for an enterprise sales rep to drive new business opportunities by qualifying and nurturing leads for the global enterprise sales team in support of our rapidly growing business. Apply here
Cofounder and Head of Product: Younger.ai, an AI Fund portfolio company, is looking for an ambitious product leader to partner with the CEO: yin to their yang. Your chief focus and responsibility will be leading design and development of a direct-to-consumer health and wellness service that helps people lead longer, more vibrant lives. Apply here
Director of Marketing & Communications: Workera, a DeepLearning.AI affiliate, seeks a hands-on marketing leader to build one of the largest AI communities in the world. In this role, you will be empowered to come up with novel ideas and programs, focusing on marketing but potentially extending to other functions as well. Apply here
Thoughts, suggestions, feedback? Please send to thebatch@deeplearning.ai. Subscribe here and add our address to your contacts list so our mailings don't end up in the spam folder. You can unsubscribe from this newsletter or update your preferences here.
Copyright 2020 DeepLearning.AI, 195 Page Mill Road, Suite 115, Palo Alto, CA 94306, United States. All rights reserved.
|