Dear friends,
Last week, I wrote about the diversity problem in AI and why we need to fix it. I asked you to tell us about your experiences as a Black person in AI or share the names of Black colleagues you admire. Thank you to everyone who responded. It was heart-warming to hear from so many of you.
Many of you shared your frustration with the lack of mentors who understand your challenges, the alienation of being the only Black face at professional meetings, and the struggle to overcome economic and social inequalities. Black women, especially, wrote about the difficulties of building a career in AI. Some of you described your efforts to support Black people in science and technology and provide tech resources to underserved communities. Thank you for sharing with us your dreams and also your disappointments.
We will feature some of your stories in our Working AI blog series. Please stay tuned.
One thing I love about the AI community is that many of us set the highest ideals for ourselves and our community — things like fairness, equity, and justice. Sometimes these ideals are so high, we may never fully live up to them, but we keep aspiring and keep trying. These days, I know it feels like society is falling far shorter of these ideals than we would like, but that's why it's more important than ever that we keep aspiring and keep trying.
It will be a long road to vanquish racism, but working together, I believe we will get there.
Keep learning!
Andrew
News
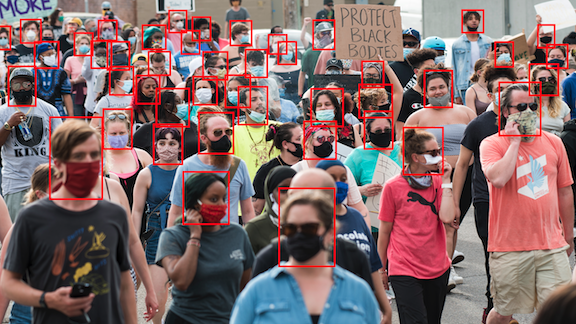
Who Was That Masked Protester?
Vendors of face recognition are updating their tech as people don masks to protect against Covid-19. Police are bound to take notice. What’s new: Companies that provide computer vision systems, including at least one that supplies law enforcement agencies, are training models to recognize obscured faces, according to USA Today. Worldwide protests in support of civil rights for Black people have energized police interest in the technology while reigniting concerns about potential violations of civil liberties. What’s happening: With people’s noses, mouths, and chins obscured by masks, companies are retraining face recognition models to identify people based only on their upper faces. Some claim to have solved the problem.
- Rank One Computing, which provides face recognition systems to 25 U.S. police forces, recently upgraded its system to identify people by eyes and eyebrows.
- SAFR, which markets to its technology schools, claims its system recognizes masked faces with 93.5 percent accuracy, but only under perfect conditions.
- U.K.-based AI firm Facewatch, which targets retail companies, says its models recognize masked individuals.
- Several municipal and federal law enforcement agencies in the U.S. have collected face imagery from protests held in recent weeks.
- In March, researchers from Wuhan University released a trio of simulated and real masked-face datasets, including one with 5,000 real-world examples. The following month, U.S.-based startup Workaround published a dataset that contains 1,200 masked selfies scraped from Instagram.
Behind the news: Many face recognition models have trouble identifying individuals even without masks, particularly members of minority groups, according to the U.S. National Institute of Standards and Technology. The agency announced plans to test the accuracy of masked face detection but suspended the effort amid the pandemic. Why it matters: Many U.S. law enforcement agencies are using face recognition to identify protesters. The questionable accuracy of these systems — particularly those aimed at masked individuals — could exacerbate the very injustices the current protests aim to highlight. We’re thinking: Face recognition technology cannot achieve its potential for good until the public can trust these systems are accurate and free of bias, both institutional and algorithmic.
Augmentation for Features
In any training dataset, some classes may have relatively few examples. A new technique can improve a trained model’s performance on such underrepresented classes. What’s new: Researchers at Jilin University, Megvii Inc., Beihang University, Huazhong University, and Tsinghua University led by Jialun Liu and Yifan Sun introduced a method that synthesizes extracted features of underrepresented classes. Key insight: The researchers trained a model and then mapped the extracted features for each data class into a two-dimensional visualization. Classes with fewer samples covered a smaller volume, making nearby decision boundaries more sensitive to variations in the features. They reasoned that artificially increasing the volume of underrepresented classes to match that of other classes should result in more robust predictions on the underrepresented classes. How it works: The researchers used well represented classes to predict the distribution of features in classes with fewer samples.
- The researchers measured the distribution of features in a given class by locating the center of all training features in that class. The distribution’s shape is defined by the variance of angles between the center and the features themselves (the tan box in the animation above).
- For each example of an underrepresented class, the researchers generated a cloud of artificial points so the cloud’s angular variance matched that of a well represented class (the yellow oval to the right of the dotted-line decision boundary above). They labeled the synthetic features as the undersampled class and added them to the extracted features.
- The network learned from the artificial features using a loss function similar to the one called ArcFace, which maximizes the distance between the center of extracted feature distributions and decision boundaries.
Results: The researchers extracted features from images using a ResNet-50. They applied those features to models built with the ArcFace loss and trained on two datasets pared down to create underrepresented classes of five examples each. Then they built models using their approach and compared the results. Their method increased the average precision (AP), a measure of true positive rate where 1 is perfect, from 0.811 AP to 0.832 AP on Market-1501. Similarly, it boosted performance from 0.732 AP to 0.742 AP on DukeMTMC-reID. Why it matters: There’s no need to generate synthetic examples if we can describe their extracted features. We’re thinking: Deep learning engineers like to use cats as examples, but these researchers focused only on the long tail.
Underwater Atlas
The ocean contains distinct ecosystems, but they’re much harder to see than terrestrial forests or savannas. A new model helps scientists better understand patterns of undersea life, which is threatened by pollution, invasive species, and warming temperatures. What’s new: Researchers from MIT and Harvard used neural networks to update existing maps of undersea ecosystems. How it works: The authors used unsupervised learning to analyze relationships between different species of plankton and the nutrients they consume.
- Drawing on data from simulations of plankton populations built by MIT’s Darwin Project, the model used a clustering algorithm to draw boundaries around areas where plankton and nutrients showed high levels of interdependence.
- The model generated a map of 115 unique ecological areas, each with a distinct balance of plankton species and nutrients.
- The researchers organized these areas into 12 ecoregions based on the life they contain. Nutrient-poor zones form aquatic deserts, while nutrient-rich areas near coastlines support biodiversity comparable to rainforests.
Results: The model’s predictions aligned well with measurements taken by scientific surveys and satellite data. Behind the news: Deep learning is being used to tackle a variety of environmental problems.
- Researchers at Austria’s University of Natural Resources and Life Sciences devised a neural network to predict harmful outbreaks of bark beetles in Germany.
- Columbia University scientists trained a model to recognize bird songs and used it to evaluate the impact of climate change on avian migration.
Why it matters: Phytoplankton feed aquatic creatures from microorganisms to whales, produce half of the world’s oxygen, and absorb enormous amounts of atmospheric carbon. Models like this could help oceanographers gauge the planet’s capacity to sustain life. We’re thinking: As educators, we're all for algorithms that help fish. We don't want them to drop out of school.
A MESSAGE FROM DEEPLEARNING.AI
Use natural language entity extraction and question-answering methods to label medical datasets. Enroll now in the AI For Medicine Specialization
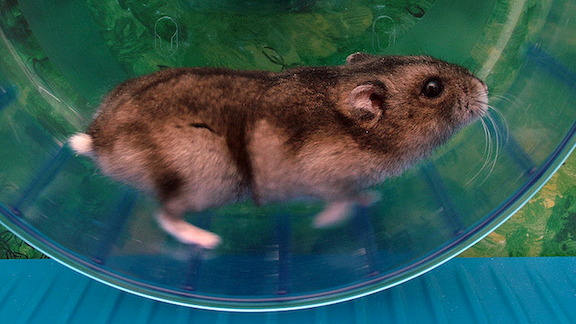
Running Fast, Standing Still
Machine learning researchers report better and better results, but some of that progress may be illusory. What’s new: Some models that appear to set a new state of the art haven't been compared properly to their predecessors, Science News reports based on several published surveys. Under more rigorous comparison, they sometimes perform no better than earlier work. Questionable progress: Some apparent breakthroughs are driven by aggregations of performance-boosting tweaks rather than core innovations. Others are mirages caused by the difficulties of comparing systems built on disparate datasets, tuning methods, and performance baselines.
- A recent MIT study found that techniques for pruning neural networks haven’t improved in a decade, despite claims that newer systems are superior. Researchers haven’t used consistent metrics and benchmarks in their comparisons, the authors said.
- A meta-analysis published in July found that recent information-retrieval models were compared using weak baselines. They didn’t outscore a model developed in 2009 on TREC Robust04, a
the bag-of-words query expansion baseline.
- A 2018 study determined that the original generative adversarial network performed as well as newer versions when it was allowed more computation to search over hyperparameters.
- The LSTM architecture that debuted in 1997 scored higher than upstart models on language modeling benchmarks once researchers adjusted its hyperparameters.
Why it matters: Machine learning can advance only through careful evaluation of earlier performance and clear measures of superiority. Erroneous claims of higher performance, even if they're unintentional, impede real progress and erode the industry’s integrity. We’re thinking: State-of-the-art approaches don't necessarily lead to better results. How hyperparameters are tuned, how datasets are organized, how models are run, and how performance is measured are also critical.
Visual Strategies for RL
Reinforcement learning can beat humans at video games, but humans are better at coming up with strategies to master more complex tasks. New work enables neural networks to connect the dots. What’s new: Ge Yang and Amy Zhang led researchers at Facebook, McGill University, and UC Berkeley to create Plan2Vec, a method that helps reinforcement learning systems strategize by representing each observation of a given task as a point on a surface. Key insight: Reinforcement learning tasks generally involve reaching a goal as efficiently as possible. If a model can represent the task at hand as a weighted graph of points in space, then a conventional planning algorithm can find the shortest path between any two points. Plan2Vec observes solutions to a maze and distorts its representation so that points on a path out are closer together. How it works: Training data for a reinforcement learning task consists of sequences of states and actions. The distance between any two states in general is not known, but the distances between states in a sequence are known.
- Plan2Vec first learns to distinguish whether or not states are neighbors using noise-contrastive estimation. This method teaches the network to mark consecutive states in a sequence as close together and non-consecutive states as far apart.
- From the predicted neighboring states, Plan2Vec extrapolates whether states from different sequences are neighbors, producing a graph that connects identified neighbors.
- A planning algorithm uses the graph to generate a continuous surface that captures the predicted distances between all states.
- To solve a task, Plan2Vec represents on the surface the starting and goal states. Then a planning algorithm finds the shortest path between them.
Results: Plan2Vec completed a 2D maze 80 percent of the time compared with a variational autoencoder (VAE) approach’s 53 percent. It solved StreetLearn, which requires navigation based on scenes along a path rather than a map, 92 percent of the time, while the VAE was successful in 26 percent of attempts. Why it matters: VAEs are good at extracting low-dimensional features from images, but the meaning of those features may not be easy to interpret. Plan2Vec creates a surface that represents how various states in a task relate to one another. This representation makes it easier to learn — and interpret — efficient solutions. We’re thinking: If we could see the strategic surface of Go, would Move 37 make sense to someone who isn’t a Grandmaster?
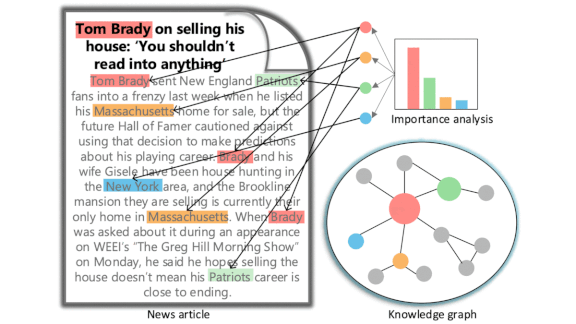
Algorithms Choose the News
Machines took another step toward doing the work of journalists. What’s new: Microsoft laid off dozens of human editors who select articles for the MSN news service and app. Going forward, AI will do the job. How it works: The tech giant declined to share details with The Batch, but recent papers published by its researchers describe methods for curating news feeds.
- A system called KRED combines a knowledge graph attention network with models for entity representation, context embedding, and information distillation. The researchers trained and tested it on nearly 1.6 million interactions between readers and news items.
- KRED also recommends local news, predicts a given article’s popularity, and classifies articles as news, entertainment, and so on. It outperformed other models on a variety of measures.
- A system called NPA matches users with news. Separate modules analyze the relevance of individual words, learn user preferences based on clicks, and score news items according to the likelihood that a given user will click on them.
- Microsoft also has AI that pairs photos with news articles. On Monday, this system matched a story about a singer's experiences of racial discrimination with a photo of her Jamaican bandmate. The company told its human editors to manually remove from its news services any articles about the misstep, The Guardian reported.
Behind the news: Other efforts to automate news curation have found ways for both machines and humans to add value.
- Apple’s News app uses algorithms to choose trending stories and fill personalized feeds while former journalists screen out fake news.
- Facebook hired editors to help curate the stories featured on its News Tab.
- Krishna Bharat, the inventor of Google News who had left the company but returned last year, has sharply criticized the service’s earlier overreliance on algorithmic recommendation.
Why it matters: In the internet era, information arrives in floods. AI could narrow that to an essential, manageable stream, but that’s a tall order when people depend on a broad range of accurate, timely news to help guide their course as individuals, communities, and societies. The Batch’s editors are thinking: Yikes!
Work With Andrew Ng
Chief Technology Officer: Rustle, an AI Fund portfolio company, seeks a CTO. You will be responsible for creating an effective way for customers and companies to interact leveraging AI, natural-language processing, and a mobile-first user interface. Apply here
Senior Full-Stack Engineer in Medellín, Colombia: Bearing AI, an AI Fund portfolio company, is looking for an experienced full-stack engineer. You will be responsible for building out the API to support our web app as well as helping to develop new AI-driven products. Contact our team directly at estephania@aifund.ai or apply here
ML Curriculum Development Director: FourthBrain.ai, an AI Fund portfolio company, is looking for a Machine Learning Curriculum Development Director to join their early stage AI Training start-up. The Director of Curriculum Development will leverage their technical skills in ML to create comprehensive project instructions, assessment and design learning experiences to support students developing their portfolio. Contact our team directly at estephania@aifund.ai or apply here
Academic Operations Director: FourthBrain.ai, an AI Fund portfolio company, is looking for an Academic Operations Director to join their early stage AI Training start-up. The Director of Operations at FourthBrain will oversee program management, ideate selection and recruiting processes for students, lead external communication, and ensure fast execution. Contact our team directly at estephania@aifund.ai or apply here
Thoughts, suggestions, feedback? Please send to thebatch@deeplearning.ai. Subscribe here and add our address to your contacts list so our mailings don't end up in the spam folder. You can unsubscribe from this newsletter or update your preferences here.
Copyright 2020 deeplearning.ai, 195 Page Mill Road, Suite 115, Palo Alto, California 94306, United States. All rights reserved.
|